In this article, we explore the combination of federated learning (FL) and meta-learning to enhance personalization in decentralized settings. FL allows data from multiple sources to be combined and learned without compromising privacy, while meta-learning enables models to adapt quickly to new data distributions. By integrating these two approaches, we can improve the efficiency, accuracy, and privacy of personalized learning systems.
To demonstrate this synergy, we conducted experiments using a real-world dataset and demonstrated improved performance compared to traditional FL methods. Our results show that combining meta-learning with FL can lead to faster convergence, enhanced communication efficiency, and better accuracy while maintaining privacy. However, there are still limitations to our study, including the reliance on a specific dataset and computational constraints.
One important limitation is that our dataset may not fully represent real-world scenarios, as it was limited by computational and time constraints imposed on us through the project. Additionally, we were unable to test the SMIL bayesian meta-learning baseline and integrate it into the federated learning framework due to complexity and AWS resource constraints.
To overcome these limitations, future research should focus on developing more diverse and representative datasets, as well as improving the efficiency and scalability of FL and meta-learning algorithms. By doing so, we can create more robust and efficient personalized learning systems that can be applied in a wide range of applications.
In conclusion, this article provides valuable insights into the potential benefits of combining federated learning and meta-learning for improved personalization in decentralized settings. By leveraging the strengths of both approaches, we can create more accurate, efficient, and privacy-preserving learning systems that can be applied in various domains. As the field continues to evolve, it is important to address the remaining limitations and push the boundaries of what is possible with FL and meta-learning.
Computer Science, Machine Learning
Optimal Meta-Learning Settings for Multi-Modal Federated Learning
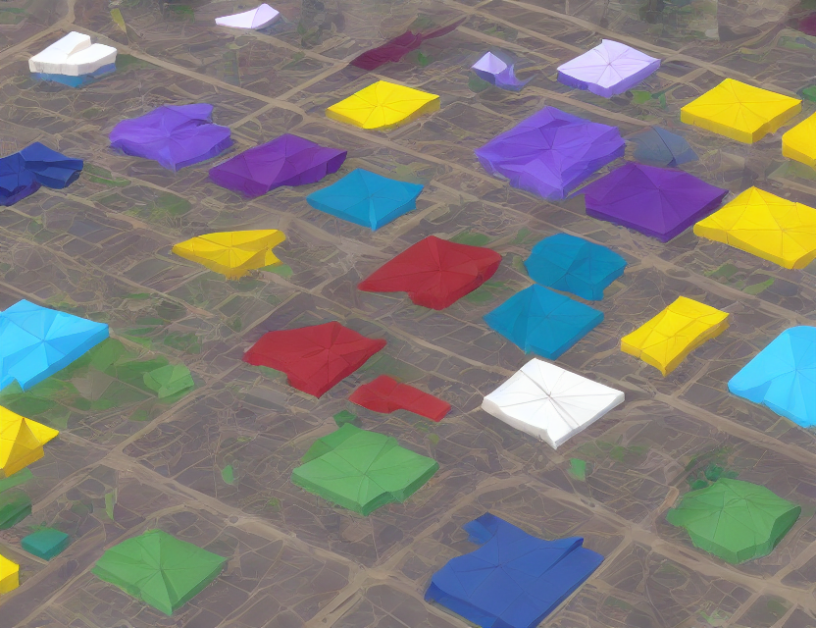