Systematic reviews (SRs) are comprehensive and rigorous assessments of existing research on a particular topic. They are crucial for making informed decisions in various fields, including healthcare, education, and business. However, conducting SRs can be time-consuming and resource-intensive due to the ever-increasing volume of published studies. To address this challenge, researchers have turned to artificial intelligence (AI) to automate certain tasks involved in SRs.
In this article, we explore how AI can be used to improve the efficiency and accuracy of SRs. We demonstrate a system that leverages techniques from generative AI and knowledge-augmented information extraction to search for relevant articles on scholarly databases. Our system can expand queries, identify additional terms and definitions, and use this context to retrieve articles more accurately.
The demo showcasing our system can be found at https://youtu.be/zMdP56GJ9mU. We also provide qualitative evaluations of our system through comparison against sentinel (ground truth) articles provided by an in-house librarian.
Our implementation involves three main steps: identifying the issue, determining the question, and writing a plan for the review (protocol). These steps are carried out by modules 1 and 2 introduced in Section 1. The remaining steps involve searching a database and using existing machine learning tools to help with later stages, including article screening, data extraction, and risk of bias assessment. This is done by module 3 in Section 1.
Our system leverages the power of generative AI, specifically ChatGPT, to expand queries and identify additional terms and definitions. We also use knowledge-augmented information extraction to search for articles more accurately. Our approach combines the strengths of both techniques to improve the efficiency and accuracy of SRs.
In conclusion, our research demonstrates the potential of AI to revolutionize the field of SRs. By leveraging generative AI and knowledge-augmented information extraction, we can reduce the time and resource burden associated with conducting SRs. Our system provides a more efficient and accurate approach to SRs, which can have significant implications for various fields.
Computer Science, Information Retrieval
Revolutionizing Systematic Reviews with Generative AI: Expanding Queries and Enriching Article Search
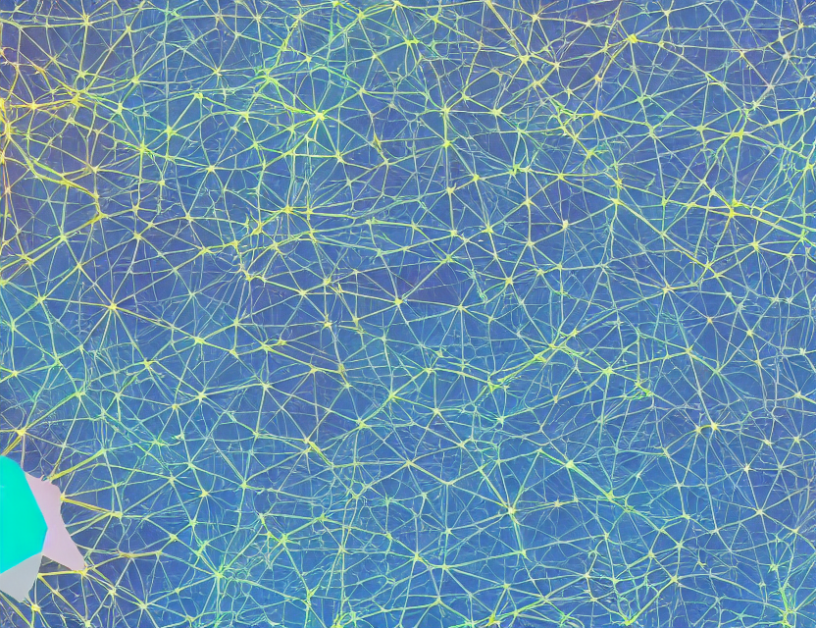