Text simplification is a crucial aspect of improving accessibility for individuals with visual impairments, as it enables faster comprehension of complex texts. However, creating models to perform this task remains challenging due to data availability issues. This study addresses these challenges by constructing a text simplification dataset specifically focusing on financial education materials. The dataset contains 5,314 pairs of complex/straightforward text segments, with the most frequent attributes being superfluous words, word length, and complex lexical expressions.
To create this dataset, six advanced philology students manually simplified text segments from four books about financial education, resulting in a total of 21 attributes requiring simplification. These attributes were identified through a histogram of the simplification rules used to generate the manually simplified dataset. The most frequent attributes, superfluous words, word length, and complex lexical expressions, were found to be the most challenging for simplification.
The dataset, including original texts, simplified versions, and identified attributes, is available online. This work provides a valuable resource for researchers working on text simplification models, particularly in specialized domains like finance. By utilizing this dataset, scientists can develop more efficient and accurate models to improve accessibility for individuals with visual impairments.
In summary, this study demonstrates the importance of creating a tailored dataset for text simplification in financial education materials, addressing the challenges of data availability and complexity. The resulting dataset provides a valuable resource for researchers working on improving accessibility through text simplification.
Artificial Intelligence, Computer Science
Simplifying Complex Texts: A Guide to Text Transformation
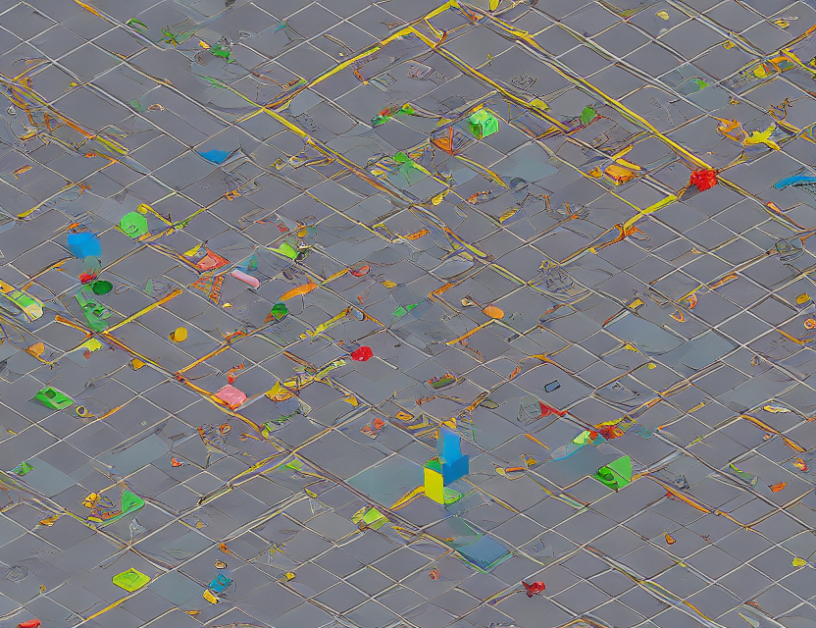