In this article, we present a new approach to multi-view stereo vision called SlimmeRF, which efficiently represents and learns the relationship between images in different views. Our method is designed to handle sparse-view scenarios, where there are few correspondences between images, by exploiting the homogeneity of components within each view.
Imagine you’re building a jigsaw puzzle with thousands of pieces. Traditional methods would require you to sort and organize all the pieces before starting the assembly process. However, our SlimmeRF method allows you to build the puzzle piece by piece, without worrying about the overall organization of the pieces. This makes it much faster and more efficient to assemble the puzzle, especially when there are many similar pieces.
Our experiments show that SlimmeRF achieves good performance in sparse-view scenarios, where other methods struggle with the lack of correspondences. Moreover, we observe that as the model is slimmed down, its performance in sparse views improves, which aligns with our hypothesis that floaters reside in components corresponding to higher ranks.
In summary, SlimmeRF is a powerful tool for efficient and accurate multi-view stereo vision, especially in sparse-view scenarios. By exploiting the homogeneity of components within each view, it reduces the computational cost and memory usage without sacrificing performance. This makes it an ideal solution for applications where efficiency is crucial, such as real-time 3D reconstruction or robotics.
Computer Science, Computer Vision and Pattern Recognition
SlimmeRF Outperforms TensorRF in NeRF-based 3D Generation
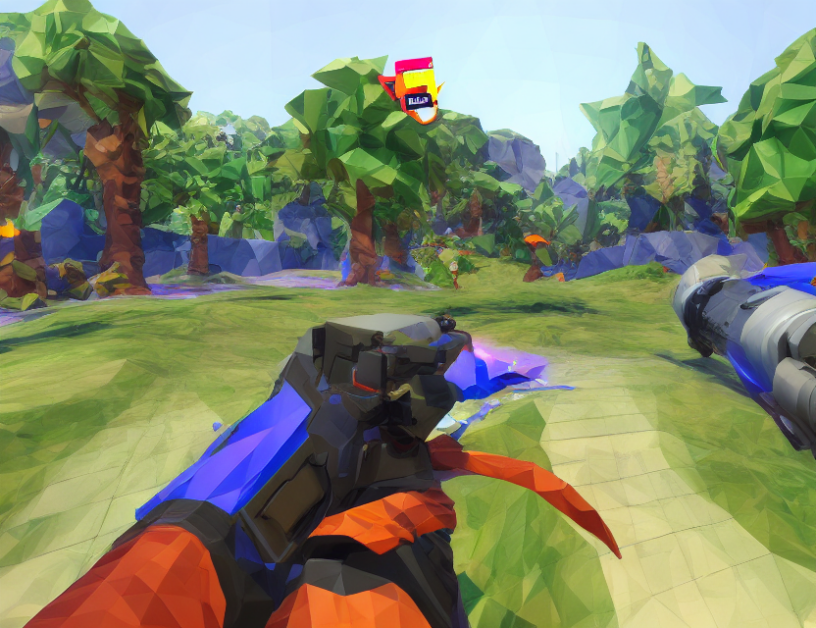