Data exploration can be a complex process, especially when dealing with large datasets. To address this challenge, conversational search tools have emerged as a powerful solution. These tools enable users to interact with data through natural language queries, allowing for more intuitive and efficient information seeking. In this article, we explore the relevance, usability, and quality of data in the context of conversational search.
Relevance: Conversational search prioritizes relevance by using natural language processing (NLP) to understand user intentions. By leveraging NLG techniques, these tools can generate summaries and visualizations tailored to users’ needs. This approach enhances the relevance of search results by providing more targeted information.
Usability: Conversational search simplifies the data exploration process by reducing cognitive load. Users no longer need to navigate through complex databases or understand complex query languages. Instead, they can interact with data through natural language queries, making it easier for them to find what they need.
Quality of Data: Conversational search also improves the quality of data by promoting a more interactive and iterative exploration process. Users can refine their searches and ask follow-up questions, resulting in more accurate and relevant data. Additionally, these tools often provide explanations and contextual information to help users better understand the data they are searching.
In summary, conversational search offers a more intuitive and efficient way to explore data by leveraging natural language processing and generation techniques. By prioritizing relevance, simplifying the process, and improving data quality, these tools can greatly enhance the user experience when working with large datasets.
Computer Science, Human-Computer Interaction
Unlocking Data Insights: A Study on Text Summarization and Hallucinations in Conversational Search
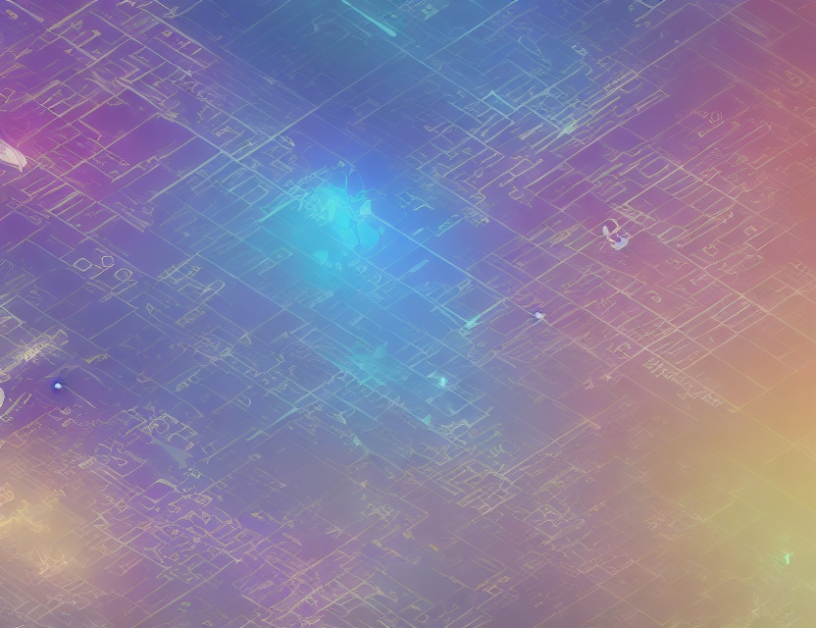