In this article, we explore a new approach to occluded person re-identification called "Pose-guided Feature Alignment." Our proposed framework leverages the Teacher-Student Decoder (TSD) to incorporate human parsing information into the Transformer architecture. This allows the model to adaptively decouple features in the absence of auxiliary supervision, resulting in improved performance.
We first provide a brief overview of the state-of-the-art methods in occluded person re-identification, highlighting their limitations and the need for innovative approaches. Next, we delve into the details of our proposed framework, including the TSD architecture and its implementation. We also discuss the key components of our method, such as the use of a masked teacher to guide the student decoder and the addition of diversity loss to improve performance.
To evaluate the effectiveness of our approach, we conduct ablation studies on two benchmark datasets: Occluded-Duke and our proposed benchmark. Our results show that our method outperforms existing state-of-the-art methods, demonstrating the superiority of Pose-guided Feature Alignment for occluded person re-identification.
Our findings have important implications for real-world applications, such as surveillance and human-computer interaction. By leveraging the power of Transformers and incorporating human parsing information into the model, we can improve the accuracy and efficiency of occluded person re-identification systems.
In summary, Pose-guided Feature Alignment is a novel approach to occluded person re-identification that leverages the Teacher-Student Decoder architecture to incorporate human parsing information into the Transformer model. Our method outperforms existing state-of-the-art methods and demonstrates the potential of this approach for real-world applications.
Computer Science, Computer Vision and Pattern Recognition
Unlocking Occluded Person Re-Identification with Attention-Aware Masks
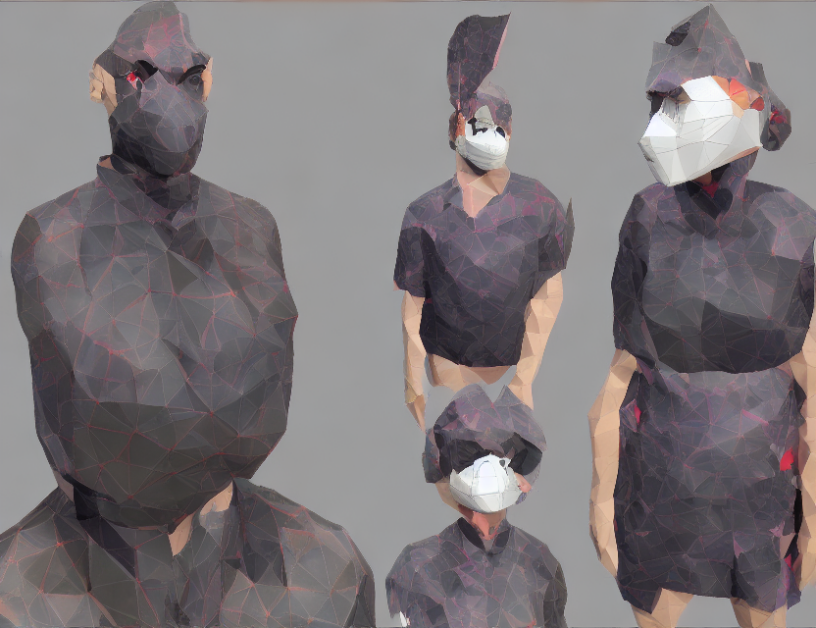