Understanding New York City’s Street Network Inference
New York City is a complex urban area with diverse neighborhoods and a vast street network. However, understanding the city’s street network can be challenging due to factors such as incomplete data or conflicting information. To address this challenge, researchers have developed new methods for inferring the city’s street network using various sources of data.
One approach is based on Bayesian inference, which allows researchers to estimate the probability distribution of the city’s street network given available data. This method can be useful when there are incomplete or missing data points, as it provides a flexible framework for incorporating prior knowledge and uncertainty. By using this approach, researchers have been able to infer the city’s street network with high accuracy, even in areas with limited data availability.
Another important aspect of understanding New York City’s street network is addressing disparities in reporting. Disparities can arise due to factors such as differences in population density or socioeconomic status, which can affect the likelihood of reporting. To address these disparities, researchers have developed new methods for quantifying spatial under-reporting disparities and identifying areas with low reporting rates. These methods can help policymakers target interventions to improve reporting and better understand the city’s street network.
In conclusion, understanding New York City’s street network is a complex task that requires careful consideration of various factors, including data availability and reporting disparities. By using innovative methods based on Bayesian inference and machine learning, researchers have been able to infer the city’s street network with high accuracy and identify areas with low reporting rates. These advances can help policymakers make more informed decisions about urban planning and infrastructure development.
Computer Science, Computers and Society
Quantifying Underreporting Disparities in Dynamic Environments
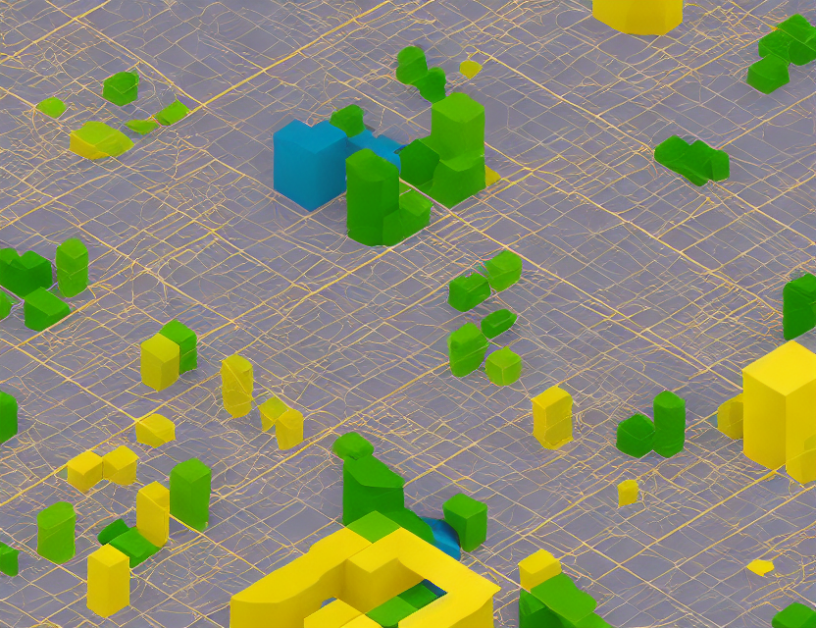