In this article, we will delve into the realm of text-conditioned generative models, specifically focusing on their applications in generating 3D shapes from text. These models have gained significant attention in recent years due to their ability to generate visually plausible and diverse 3D objects based solely on textual descriptions. We will explore the different approaches used to achieve this feat, including the popular Score Distillation Sampling (SDS) method, which leverages image-space guidance to create more realistic 3D shapes.
To better understand how these models work, let’s consider an analogy: Imagine you have a recipe book filled with different types of dishes, each described in written instructions. Using this book, you can conjure up any dish you want by following the provided instructions, much like how text-conditioned generative models create 3D shapes from textual descriptions.
One popular approach for generating 3D shapes is through the use of neural radiance fields (NeRFs). These models combine the power of deep learning with traditional mesh-based head modeling to generate volumetric hairstyles that are both realistic and varied. However, current methods only capture the outer visible surface of the 3D shape, lacking a meaningful internal hair structure.
To overcome this limitation, researchers have turned to image-space guidance techniques like SDS. By leveraging text-to-image generative diffusion models, such as Stable Diffusion [42], these methods can create more detailed and realistic 3D shapes by incorporating the textual description into the generation process. This approach has gained popularity in recent years due to its ability to produce high-quality results that align with the provided textual description.
In summary, text-conditioned generative models have revolutionized the field of computer graphics and computer vision by enabling the creation of 3D shapes from textual descriptions. While current methods have their limitations, advancements in techniques like SDS hold great promise for generating more realistic and detailed 3D assets in the future. By understanding the underlying mechanics of these models, we can unlock new possibilities for creating virtual worlds and characters that are both visually plausible and imaginative.
Computer Science, Computer Vision and Pattern Recognition
Unlocking the Potential of Diffusion Models for Text-to-3D Hair Synthesis
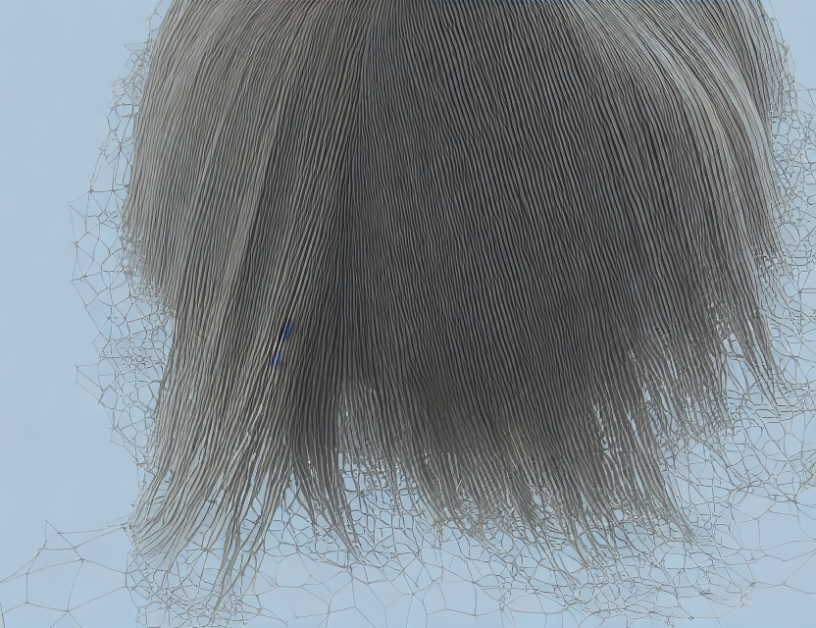