In this article, the authors present their new model for end-to-end text-to-speech (TTS) synthesis called FastSpeech 2. They aim to produce high-quality speech with fast conversion times. The model uses a combination of attention mechanisms and multi-resolution representations to effectively process both short and long-range dependencies in the input text.
The authors explain that previous TTS models have limitations, such as slow conversion times and lack of emotional expressiveness. They propose their new model as an improvement over these models, as it can generate speech at a faster rate while maintaining high-quality output.
To evaluate their model’s performance, the authors conduct objective and subjective experiments using several benchmark datasets. The results show that FastSpeech 2 outperforms all state-of-the-art baselines in terms of emotional expressiveness.
The article also discusses related works in the field of TTS, highlighting the challenges of incorporating multi-modal information into NLP models. The authors note that while some studies have explored the use of external commonsense knowledge for emotion recognition, these approaches are limited by their reliance on pre-defined rules and lack of consideration for the context in which the speech is being generated.
Overall, FastSpeech 2 represents a significant advancement in TTS technology, offering faster and more emotively expressive speech synthesis capabilities. The authors’ approach demonstrates the potential for improving the quality and efficiency of TTS systems, with implications for a wide range of applications, from voice assistants to language learning tools.
Computation and Language, Computer Science
Emotion Intensity Quantization for Fine-Grained Controllable Emotional Text-to-Speech
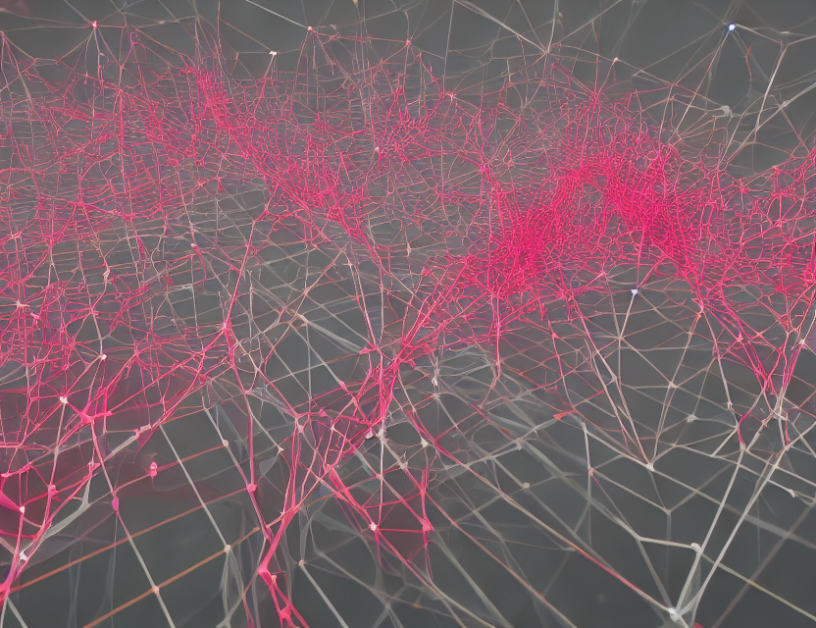