Long-horizon manipulation tasks are crucial for robotics systems to perform complex tasks autonomously. These tasks require both low-level control policies and high-level planning capabilities. The article presents a dataset called LHManip, which offers the opportunity to develop robots that can learn and adapt to novel environments without being explicitly programmed for each task.
The dataset includes natural language instructions for solving various manipulation tasks in cluttered tabletop environments. For instance, the task "Clean the pan" requires the robot to pick a sponge and perform a movement on the pan, while the task "Cook the capsicum" involves placing it in the pan and then on a plate.
The authors believe that the robot must be able to infer necessary information from the natural language instruction without any additional low-level environment descriptions. This approach enables the development of robots with high-level reasoning capabilities, allowing them to solve complex tasks in novel environments.
To summarize, LHManip provides a valuable dataset for developing robots that can perform long-horizon manipulation tasks autonomously and adapt to new settings without explicit programming. The dataset includes natural language instructions for various tasks, requiring the robot to infer necessary information from the instruction alone. This approach enables the development of more advanced and capable robots in the future.
Grounded Manipulation in Cluttered Environments with Multi-Task Transformer
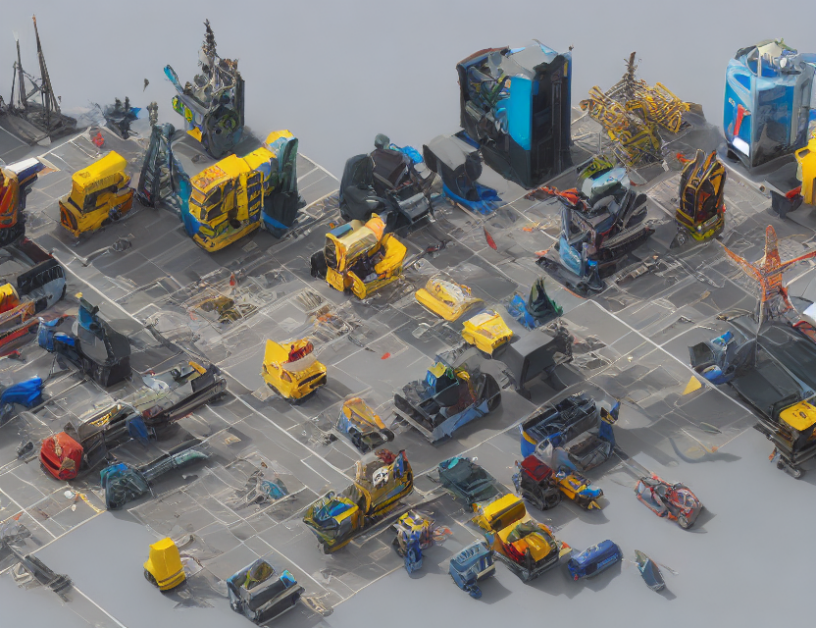