In this article, we propose a novel approach for grasping objects with robotic arms. Our method combines physics-based simulation and machine learning to accurately predict the outcome of grasping actions. We evaluate our approach through extensive experiments using mesh models provided by other researchers and show significant improvements in success ratio and clear ratio compared to existing methods.
To understand how our approach works, let’s break it down into simpler terms. Imagine you are trying to pick up a ball with your hand. The distance between your hand and the ball affects the likelihood of successfully grasping it. If you are too far away, the ball may roll away or fall. If you are too close, you may drop it. Our approach takes into account this relationship between distance and success by using physics simulation to accurately model the motion of the robotic arm and the object being grasped.
We also use machine learning algorithms to classify the object’s boundaries based on its shape and size. This helps to filter out invalid grasps, such as those made inside the object, ensuring that only successful grasps are counted towards our success ratio and clear ratio metrics.
To test the effectiveness of our approach, we conducted extensive experiments using mesh models provided by other researchers. Our results show a significant improvement in both success ratio and clear ratio compared to existing methods. This means that our approach is more accurate and efficient than previous methods, making it ideal for real-world applications.
In conclusion, our proposed approach for grasping objects with robotic arms combines physics-based simulation and machine learning to accurately predict the outcome of grasping actions. Through extensive experiments, we demonstrate significant improvements in success ratio and clear ratio compared to existing methods, making our approach more effective and efficient than previous approaches. By using this approach, robots can successfully grasp objects with a high degree of accuracy and reliability, opening up new possibilities for robotic manipulation in various industries.
Improved Grasping via Weighted Power Grasps
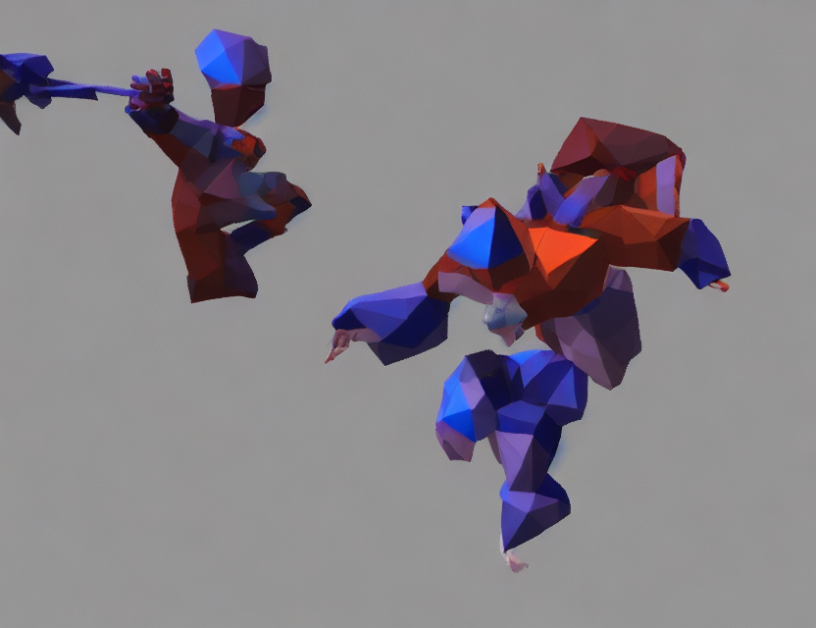