Gemini is a family of artificial intelligence models designed for various tasks, including summarization, reading comprehension, and multilingualism. These models are highly capable and engineered for on-device deployments, allowing them to perform exceptionally well in tasks such as factuality retrieval, coding, math/science, and reasoning. The models are trained on a sequence length of 32,768 tokens and use long filler text to effectively utilize their context length.
The authors conduct a synthetic retrieval test to verify that the Ultra model can retrieve the correct value with 98% accuracy when queried across the full context length. They also plot the negative log likelihood (NLL) versus the token index across a held-out set of long documents, showing that the NLL decreases with sequence position up to the full 32K context length.
Gemini’s ability to utilize long context lengths enables new use cases such as retrieval over documents and video understanding. These models can be used for various applications, including image recognition at scale, reading comprehension requiring discrete reasoning over paragraphs, and multilingual evaluation of 128 languages.
In summary, Gemini is a powerful family of AI models capable of handling complex tasks with ease, thanks to their ability to utilize long context lengths. These models can be used in various applications, including image recognition, reading comprehension, and multilingualism, making them highly versatile and valuable tools for any AI enthusiast or professional.
Computation and Language, Computer Science
Machine Translation Advances: Large Language Models and Extreme Summarization
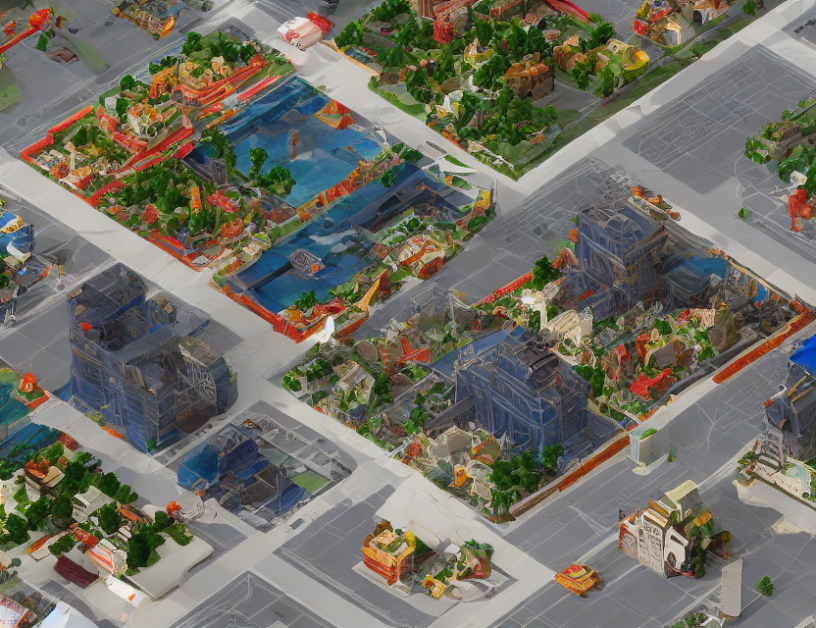