In this article, we explore the concept of Semantic VAD, a novel approach to voice activity detection (VAD) that leverages deep learning techniques to improve accuracy and efficiency. Traditional VAD methods rely on simple binary classification, but Semantic VAD takes it to the next level by incorporating semantic information into the model. This allows the system to better understand the context of the speech, leading to improved performance in noisy environments.
To create a Semantic VAD system, we build upon the DFSMN (Deep Fusion of Spectral and Mel-Spectral Features with Multi-Task Learning) architecture, which has shown significant improvements over traditional methods. We then integrate two enhancements: RWKV (Reinventing RNNs for the Transformer Era) and SAN-M (Memory Equipped Self-Attention for End-to-End Speech Recognition). These additions enable the system to better handle complex speech scenarios, such as multi-speaker interactions.
One of the key advantages of Semantic VAD is its ability to adapt to various environments and scenarios. This is achieved through a novel attention mechanism that can focus on different parts of the input signal in real-time. This allows the system to respond more effectively to changing conditions, such as background noise or speaker interruptions.
Another important aspect of Semantic VAD is its ability to improve over time. By incorporating feedback from users and continuously updating the model, we can optimize performance and reduce errors. This ensures that the system remains effective in real-world scenarios, where conditions can be unpredictable and constantly changing.
Overall, Semantic VAD represents a significant advancement in voice activity detection technology. By leveraging deep learning techniques and integrating improved attention mechanisms, we can create systems that are more accurate, efficient, and adaptive to changing environments. As the field of speech interaction continues to evolve, we can expect to see even more innovative approaches like Semantic VAD shaping the way we interact with technology.
Semantic VAD: Low-Latency Voice Activity Detection for Speech Interaction
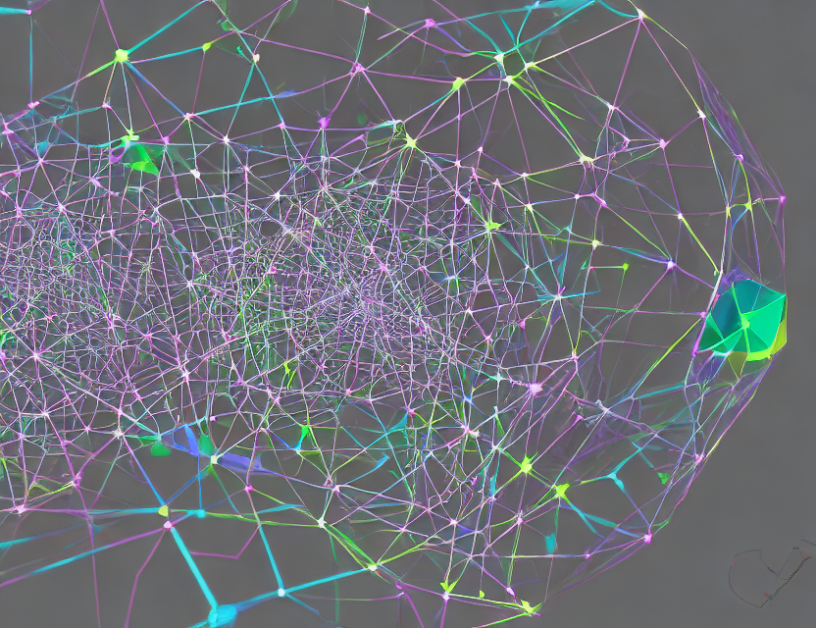