This article discusses the potential of Large Language Models (LLMs) to revolutionize various fields such as chat agents, language translation, and general queries. LLMs are based on Transformer-based Deep Learning models that can summarize and predict new content based on provided context. These models have been trained on billions of texts and can semantically organize the learned text to provide answers more efficiently than humans. However, generating output for a focused set of activities is complex due to the models’ generalized nature over large text datasets with various topics.
The article highlights recent advances in Natural Language Processing (NLP) through LLMs and their potential applications. These include language translation, sentiment prediction, and new procedures not hardcoded in the script. The authors emphasize that while LLMs can perform tasks more efficiently than humans, they are not perfect and may generate oversimplified or non-existent details (Wen et al., 2023).
To better understand the capabilities of LLMs, the article examines their limitations and challenges. For instance, these models can provide answers that lack the nuances and complexities of human language use. Additionally, integrating virtual mediums and interviews to study human behaviors has been inconsistent due to the diversity and complexity of these encounters (Lindsay & Norman, 2013).
The authors also discuss the potential of LLMs for conversation as chat agents and their ability to perform new procedures not hardcoded in the script (Andreas, 2022). They acknowledge that while these models have shown promising results, they are still being tested by the research community.
In conclusion, this article demonstrates how LLMs can revolutionize various fields by providing efficient and accurate summaries and predictions. However, these models also face limitations due to their generalized nature, and further research is needed to overcome these challenges.
Computer Science, Computers and Society
Advances in Generative Agents Driven by Large Language Models: A Survey
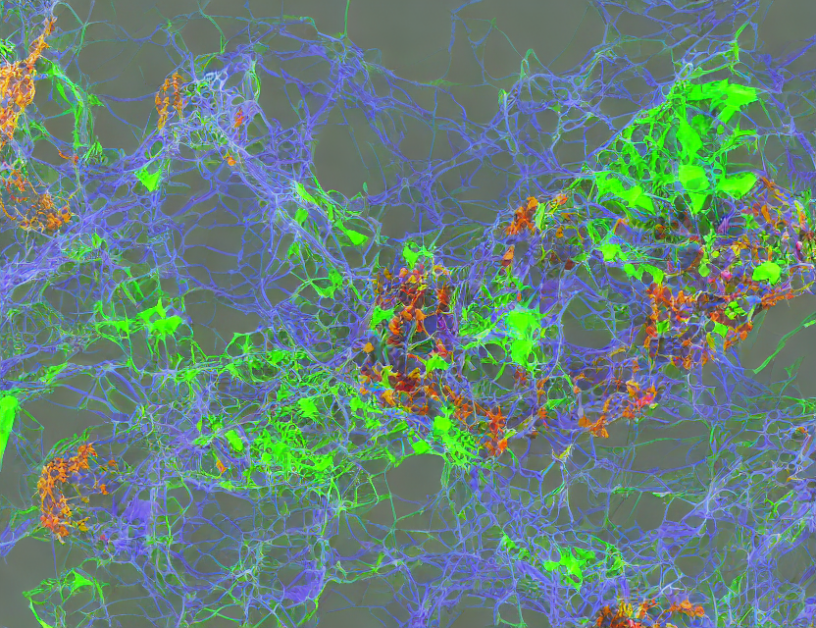