In this paper, the authors propose a novel approach to address the subsection rupture problem in vessel-like structure segmentation tasks using graph convolutional networks (GCNs) and refinement segmentation methods. They argue that previous approaches have neglected the integrity of vessel-like structures and the particularity of the subsection rupture problem, leading to poor performance in addressing this issue.
To tackle the subsection rupture problem, the authors propose a deep network-based refinement segmentation method that leverages GCNs to learn feature representations for each subsection rupture and improve their accuracy. They design various feature learning modules and loss constraints to optimize the performance of the deep network, while ensuring the integrity of vessel-like structures is preserved.
The authors evaluate their proposed approach on several public datasets and demonstrate its effectiveness in addressing the subsection rupture problem compared to existing methods. They also analyze the results of various feature learning modules and loss constraints to provide insights into the performance of their method.
In summary, this paper presents a novel approach to addressing the subsection rupture problem in vessel-like structure segmentation tasks using GCNs and refinement segmentation methods. By leveraging these advanced techniques, the authors are able to improve the accuracy of subsection rupture segmentation while preserving the integrity of vessel-like structures. This work has important implications for medical image analysis and could potentially lead to improved diagnosis and treatment of vascular diseases.
Computer Science, Computer Vision and Pattern Recognition
Enhancing Confidence Calibration in Semantic Segmentation with Refinement Networks
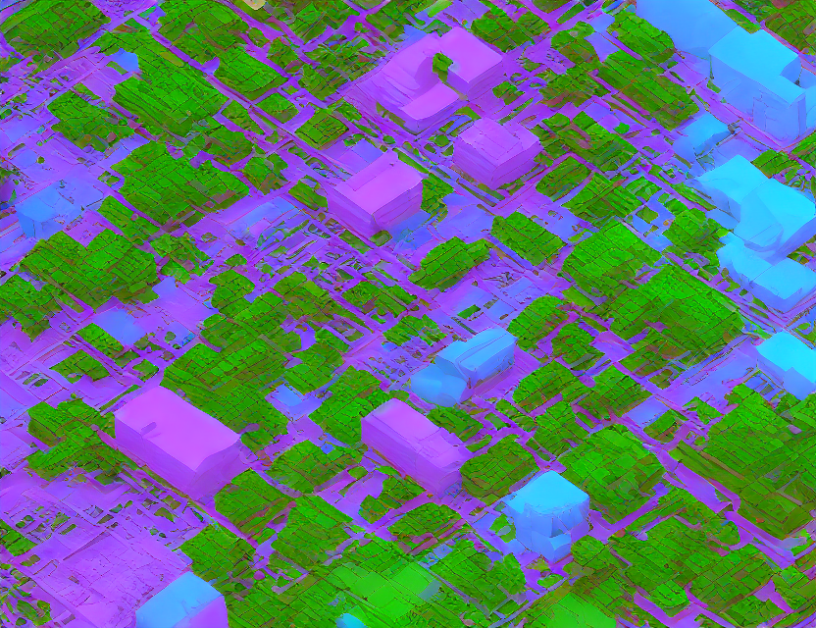