Video understanding is a critical aspect of artificial intelligence, with applications in various industries, including entertainment, security, and healthcare. However, developing a comprehensive understanding of videos remains a challenging task due to their complex nature, which involves multiple labels and untrimmed videos. To address this issue, researchers proposed a new framework called Trans-Temporal Multi-Label Learning (TTML).
The TTML approach leverages the power of pretext tasks to learn rich features from raw video data. By combining these features with temporal relationships, the model can predict multiple labels for each frame in a video. This process enables the framework to capture the context and dependencies between different frames, resulting in improved accuracy compared to traditional methods.
To evaluate the effectiveness of TTML, the authors conducted experiments on several datasets, including HVU, which is a large-scale dataset with various labels for multi-label videos. The results demonstrated that TTML outperformed the previous state-of-the-art method by 1.3%, indicating its superiority in real-world scenarios.
In summary, TTML is a promising framework that simplifies video understanding by leveraging pretext tasks and temporal relationships. By combining these techniques, the model can predict multiple labels for each frame in a video, leading to improved accuracy compared to traditional methods. The effectiveness of TTML is demonstrated through experiments on several datasets, including HVU, which shows its potential in real-world scenarios.
Computer Science, Computer Vision and Pattern Recognition
Enhancing Temporal Pretext Tasks with Local Patch Augmentations: A Novel Approach to Improve Downstream Feature Performance
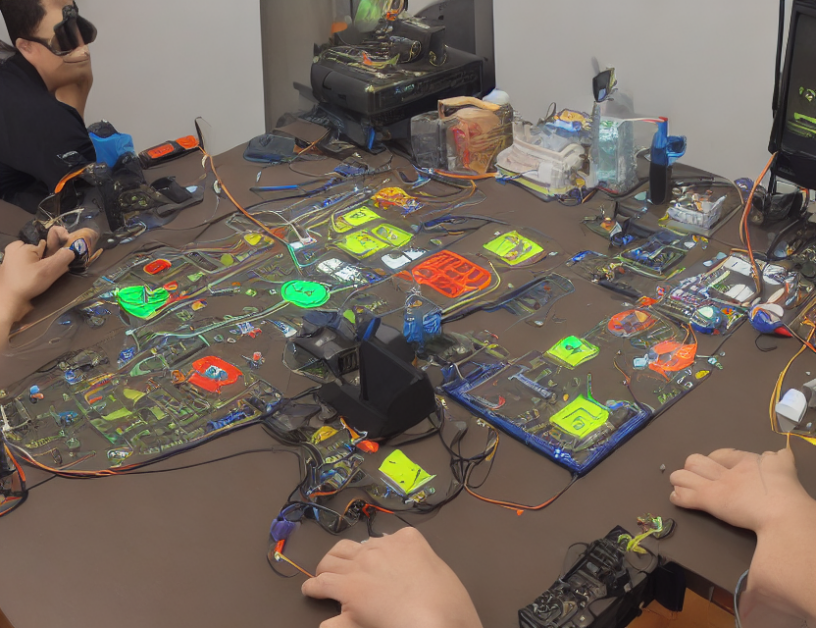