In this article, the authors propose a novel approach to image editing called Fairy, which leverages the power of diffusion-based multimodal fusion. They aim to create an efficient and flexible method that can handle various editing tasks with high quality and temporal consistency. The key innovation lies in the use of anchor-based attention, which allows the model to focus on specific frames or regions while minimizing feature disparity between them. This approach enables Fairy to scale to arbitrary long videos without suffering from memory issues.
The authors begin by highlighting the limitations of traditional image editing methods, which often result in inconsistent quality and unnatural-looking results. They argue that these shortcomings can be overcome by combining multiple modalities, such as visual and textual information, to create a more comprehensive understanding of the input data. Fairy addresses this challenge by fusing these modalities through diffusion-based fusion, which enables the model to capture long-range dependencies and contextual relationships between frames or regions.
To improve efficiency and flexibility, Fairy employs an anchor-based attention mechanism. This allows the model to selectively focus on specific frames or regions while processing the remaining content. The authors demonstrate that this approach significantly reduces the computational complexity and memory requirements compared to traditional methods.
The article proceeds to present a series of experiments that validate the effectiveness of Fairy in various image editing tasks, such as stylization, arbitrary long videos, and input faithfulness. The results show that Fairy outperforms existing methods in terms of quality, consistency, and efficiency. The authors also analyze the performance of Fairy in different scenarios, including stylization, where it can recognize various styles while maintaining high-quality output, and arbitrary long videos, where it can scale without sacrificing temporal consistency.
The authors conclude by emphasizing the potential applications of Fairy in a wide range of industries, from entertainment to advertising and healthcare. They believe that their approach represents a significant breakthrough in the field of image editing and content creation, enabling creators to produce high-quality content efficiently and flexibly.
In summary, Fairy is a novel image editing approach that leverages diffusion-based multimodal fusion and anchor-based attention to enable efficient and flexible editing of images. The authors demonstrate its effectiveness in various tasks, including stylization, arbitrary long videos, and input faithfulness, and highlight its potential applications in multiple industries.
Computer Science, Computer Vision and Pattern Recognition
Enhancing Video Editing Efficiency with Temporal Attention
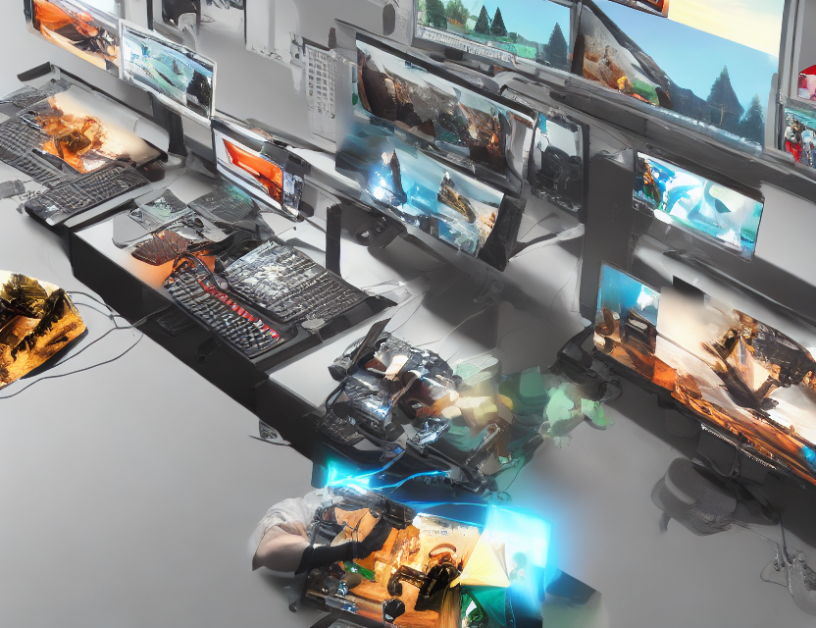