In this research paper, the authors aim to address the challenges associated with semantic data, which is inherently diverse and varies across different sources. They propose a novel approach called Semantic Encoder-Enhanced Representation (SEER), which leverages diverse semantic sources such as word2vector (W2V), logic-explained network features (LEN), hand-annotated semantics (SA), or other relevant resources. The SEER model extracts adaptable latent spaces across multiple visual and semantic features, covering both seen and unseen data.
The authors emphasize the importance of gaining precise control over semantic information within these latent spaces while minimizing non-relevant details in the domain. They achieve this by using various techniques such as dimensionality reduction and feature learning to ensure that the model can adapt to different scenarios. The SEER model is tested on several datasets, including W2V and visually grounded semantic annotations (VGSE), and shows improved performance compared to existing approaches.
To demystify complex concepts in the article, let’s use everyday language and metaphors to help readers understand the key ideas. For instance, the authors explain that the SEER model is like a toolbox for working with diverse semantic data. Just as a toolbox contains various tools for different tasks, the SEER model has different components that work together to handle the complexities of semantic data.
In summary, the article presents a novel approach to tackling the challenges associated with semantic data by proposing the SEER model. This model leverages diverse sources of semantic information to create adaptable latent spaces that can be used across various visual and semantic features. The authors demonstrate the effectiveness of the SEER model on several datasets, showing improved performance compared to existing approaches. By using simple language and engaging metaphors, readers can easily grasp the key concepts of this groundbreaking research.
Computer Science, Computer Vision and Pattern Recognition
Generalized Zero-Shot Learning: A Comprehensive Review
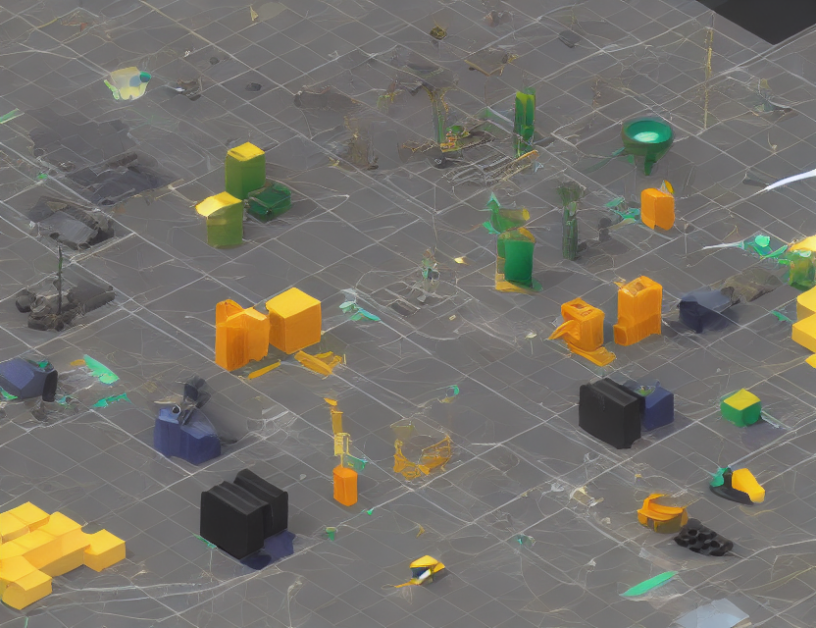