Adversarial attacks have become a significant concern in machine learning, threatening the robustness of neural networks. In this context, Yasaman Bahri et al.’s article "Explaining Neural Scaling Laws" sheds light on the underlying mechanisms governing adversarial robustness. By delving into the neural scaling laws that govern these systems, the authors provide valuable insights into improving adversarial robustness.
The article begins by acknowledging the pressing need to address the environmental impact of artificial intelligence (AI) and its carbon footprint. The authors highlight the importance of prioritizing efficiency as a cardinal metric alongside accuracy to mitigate AI’s environmental impact and foster inclusivity. They emphasize that similar studies on adversarial robustness are nearly absent in this context, prompting their pioneering exploration into neural scaling laws.
To explain these laws, the authors employ metaphors from physics, comparing neural networks to physical systems that scale with size. By understanding the underlying mechanisms driving these scaling laws, they demonstrate how to improve adversarial robustness. The article presents a novel approach to generating adversarial examples using generated data, showcasing its effectiveness in improving robustness.
The authors emphasize that their work is part of a broader effort to prioritize efficiency and address the environmental impact of AI. They acknowledge the contributions of other researchers in this area, including those exploring carbon accountability in AI. The article concludes by underscoring the importance of balancing accuracy with efficiency in mitigating adversarial attacks.
In summary, Bahri et al.’s article offers a groundbreaking investigation into neural scaling laws and their implications for improving adversarial robustness. By demystifying complex concepts through analogies and metaphors, they provide valuable insights into the mechanisms governing these systems, paving the way for more efficient and robust AI.
Computer Science, Machine Learning
Improving Robustness in Deep Learning Models: A Generated Data-based Approach
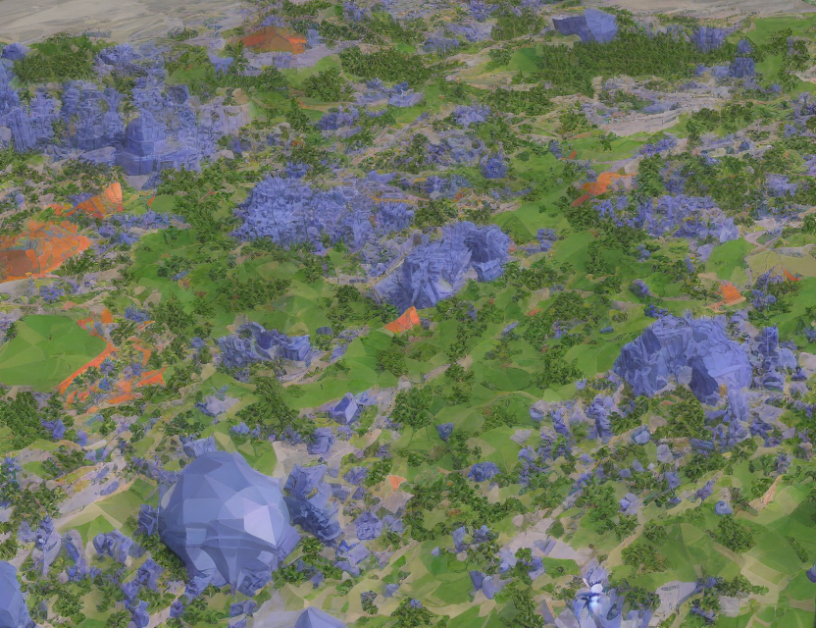