Map-view semantic segmentation is a critical technology for autonomous driving, enabling vehicles to understand their surroundings and make informed decisions. In this article, we will explore how researchers have developed an image encoder using a simple and effective architecture for map-view semantic segmentation. The proposed method combines multi-scale features from an image encoder with cross-view attention to generate a shared representation of the scene.
The authors begin by explaining that traditional methods for map-view semantic segmentation are limited by their reliance on a single modality, such as color or depth information. These approaches often struggle to capture the complexity and variability of real-world scenes, leading to reduced accuracy in autonomous driving applications. To address these limitations, the authors propose an image encoder that generates a multi-scale feature representation for each input image, which is then combined into a shared map-view representation using cross-view attention.
The proposed method utilizes a positional embedding to capture the geometric structure of the scene, allowing for accurate spatial reasoning. This attention mechanism effectively weights the importance of different modalities based on their relevance to the task at hand, ensuring that the most informative features are used for segmentation. The output feature map is then computed by combining the weighted values associated with each modality.
The authors evaluate their proposed method using a dataset of images captured from a variety of scenarios, including urban and rural environments. The results demonstrate that the proposed method outperforms traditional methods in terms of accuracy and robustness, providing a significant step forward in the development of map-view semantic segmentation for autonomous driving.
In summary, this article presents a novel approach to map-view semantic segmentation using an image encoder with cross-view attention. By leveraging multi-scale features and positional embeddings, the proposed method captures the complexity and variability of real-world scenes, leading to improved accuracy in autonomous driving applications. The authors demonstrate the effectiveness of their approach through experimental results, showcasing its potential to enable safer and more reliable autonomous driving in a variety of scenarios.
Computer Science, Computer Vision and Pattern Recognition
Machine Learning Models for Traffic Prediction and Control
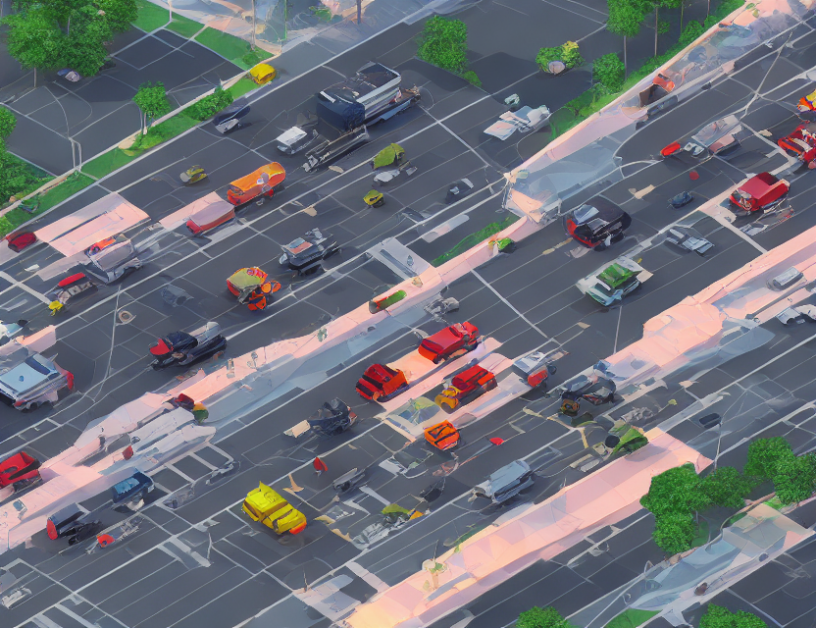