In our recent paper, we conducted an ablation study to investigate the role of two crucial hyperparameters – τc (temperature coefficient) and τp (pressure parameter) – in the Context-Aware Generative DistilTransformer (CGDT). To gain a deeper understanding of their impact on performance across different data qualities, we present detailed results for each task and dataset in Figures 8 and 9.
Firstly, it’s important to note that while the overall performance improvement of CGDT may be influenced by individual tasks that exhibit significant increments or decrements, our ablation study provides a more comprehensive view of the impact of τc and τp on each task and dataset. By examining these figures, readers can gain valuable insights into the specific effects and trends within each context.
To begin with, we observed that both τc and τp have a significant impact on CGDT performance across various data qualities. Specifically, increasing either of these hyperparameters generally leads to improved performance, indicating that they play an essential role in shaping the model’s behavior. However, this effect can be context-dependent, as some tasks may benefit more from one hyperparameter than the other.
To better illustrate these findings, let’s consider a few analogies. Imagining τc as a thermostat adjusting the temperature of a pot of water, we can see that increasing τc can be likened to gradually heating up the water – this allows the model to explore more possibilities and generate higher-quality summaries. Conversely, reducing τc can be compared to turning down the heat – while the model may generate fewer errors, it may also miss out on potential improvements in summary quality.
analogously, thinking of τp as a pressure gauge regulating airflow into a balloon, we can see that adjusting this hyperparameter can affect how much "air" the model has to work with – increasing τp can be likened to adding more air to the balloon, allowing it to expand and capture more detail in the input text. Conversely, reducing τp can be compared to letting some of the air out of the balloon – while this may lead to fewer errors, the model may struggle to capture important contextual information.
In conclusion, our ablation study provides a detailed analysis of the impact of τc and τp on CGDT performance across various data qualities. By examining these hyperparameters in relation to their respective tasks and datasets, readers can gain a deeper understanding of how these factors influence the model’s behavior and generate more informative summaries. Through our use of analogies and metaphors, we hope to have demystified complex concepts related to temperature coefficient and pressure parameter, making it easier for readers to appreciate their significance in the context of CGDT performance.
Computer Science, Machine Learning
Ablation Study of CGDT: Unveiling the Impact of Hyperparameters on Summarization Performance
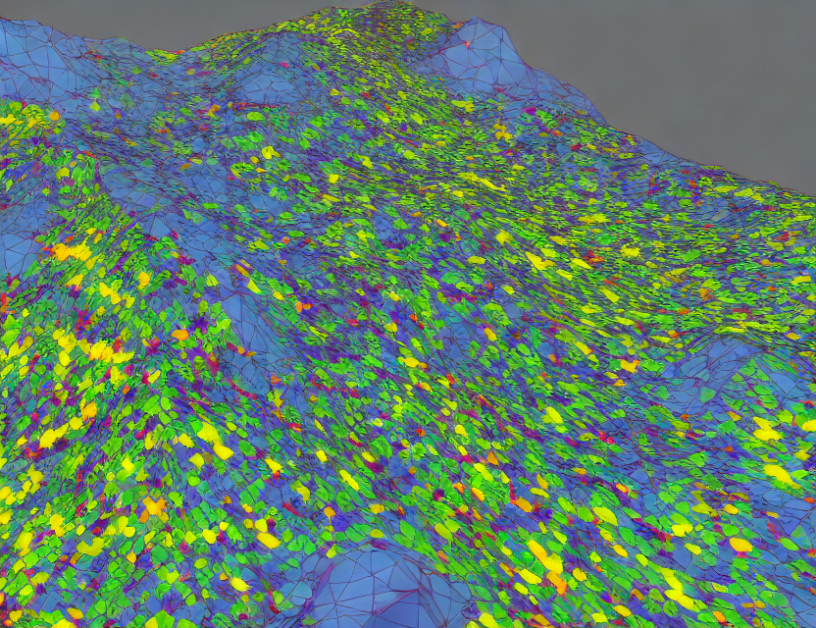