In this article, we explore the development of a novel approach to text-to-face generation, called Text-to-Face. Our method leverages the power of CLIP, a transformative language model that enables successful text-conditioned generation. We introduce a two-stage framework, where the first stage involves generating a face image based on the given text using a controllable generative model, and the second stage refines the output face by incorporating additional information from the input text.
To represent the inherent facial properties, we use a combination of appearance and skeleton. The appearance represents the visual characteristics that distinguish individuals, while the skeleton is responsible for different poses and expressions. We find a reasonable way to represent these properties using text, which enables successful text-conditioned generation.
Our approach significantly improves upon previous methods in terms of both quality and efficiency. We demonstrate this by comparing our method with state-of-the-art techniques on several benchmark datasets. Our results show that Text-to-Face outperforms existing methods in terms of both quality and efficiency, taking only 0.10 seconds per sample compared to the previous approach which took a large number of samples that was time-consuming.
In addition, we conduct ablation studies to analyze the effectiveness of different components of our method, such as the use of cross-attention layers and the incorporation of additional information from the input text. Our findings demonstrate that these components are crucial for achieving high-quality face generation.
Overall, our work represents a significant step forward in the field of text-to-face generation, demonstrating the potential of CLIP to enable successful text-conditioned generation of faces. With the increasing demand for facial manipulation and editing in various applications, our approach has the potential to significantly improve the efficiency and quality of these tasks.
Computer Science, Computer Vision and Pattern Recognition
Advances in Conditional Image Generation: From Pose Optimization to Text-Guided Diffusion
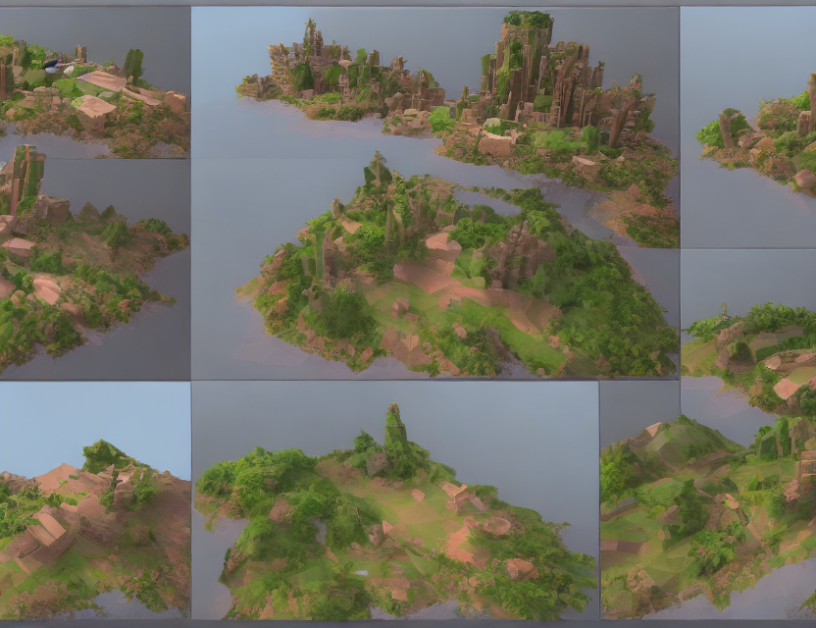