In this article, we explore the concept of emphasis transfer in machine translation, specifically focusing on two types of models: Seamless M4T and human evaluation. Emphasis transfer refers to the ability of a model to accurately convey the intended emphasis of a source language utterance into a target language one.
To evaluate these models, we created a comprehensive framework that includes both automatic and human evaluations. For the automatic portion, we developed a classifier that can detect which word(s) are emphasized in an output utterance based on its word-tokenized transcription. We then used word-to-word alignment to identify the correct word(s) to emphasize in the output utterance.
Our human evaluation involved conducting an annotation task with expert annotators who were presented with an utterance and its word-tokenized transcription. They marked which words they considered emphasized, and their annotations were used to compute precision, recall, and F1 scores for English-to-English and English-to-Spanish models.
The results show that Seamless M4T model performs poorly in capturing emphasis, yielding an F1 score of 14% in English-to-Spanish translation. In contrast, human annotators were able to accurately identify the emphasized words in the output utterance with a high degree of precision.
Overall, this study demonstrates the importance of comprehensive evaluation methods for assessing the quality of emphasis transfer in machine translation. By combining both automatic and human evaluations, we can gain a more complete understanding of how well a model is able to convey the intended emphasis of a source language utterance into a target language one.
Computation and Language, Computer Science
Assessing Emphasis in Multilingual Speech Translation: A Comparative Study
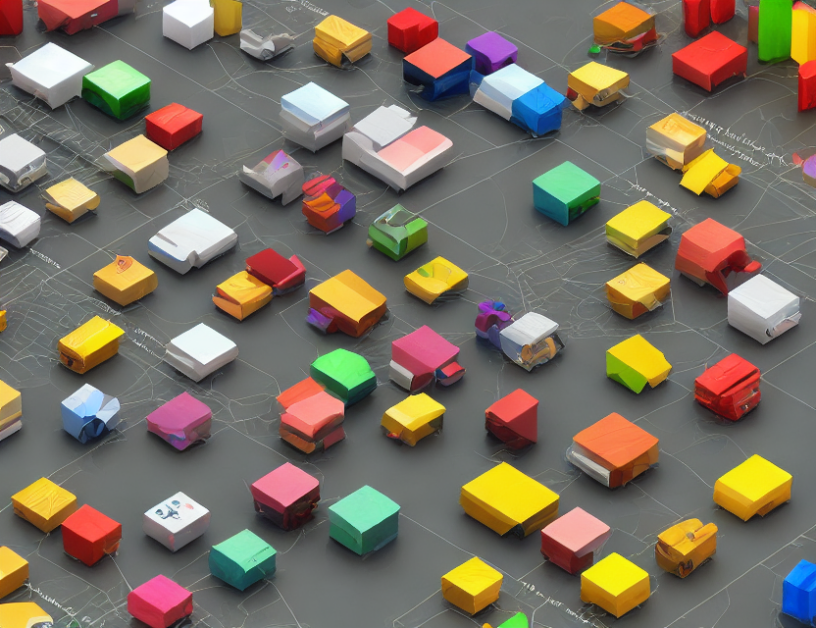