In this article, the authors propose a novel deep learning model called Attention-based Two-handed MANO (ATM) to learn hand gestures from video data. The ATM model is designed to address two main challenges in hand gesture recognition: 1) joint localization and 2) hand pose estimation.
To tackle the first challenge, the authors utilize a novel attention mechanism that focuses on the most relevant body parts for each hand. This allows the model to accurately locate the hands in the video frame. The attention mechanism is inspired by the human brain’s attentional mechanisms, which allow us to selectively focus on specific stimuli while ignoring others.
To address the second challenge, the authors incorporate a two-handed MANO (Multi-output Atlas Network Output) model that can learn both hands simultaneously. This allows the model to capture the complex relationships between the hands and the surrounding context in each video frame. The MANO model is similar to a piano player’s two hands working together to play a beautiful melody – each hand plays its own unique role, but they must work together in harmony to create the final product.
The ATM model consists of several components, including a feature extractor, an attention module, and a MANO model. The feature extractor generates a set of hand-related features from each video frame, such as shape, pose, and movement. The attention module then focuses on the most relevant features for each hand, much like how we selectively attend to specific sounds or smells in our environment. Finally, the MANO model combines the attended features to estimate the 3D hand pose of both hands.
The authors evaluate the ATM model on several challenging datasets and demonstrate its superior performance compared to existing state-of-the-art methods. They also show that their model can generalize well to unseen data, which is critical for real-world applications where hand gesture recognition may encounter diverse and dynamic environments.
In conclusion, the ATM model represents a significant advancement in the field of hand gesture recognition. By combining attention mechanisms with two-handed MANO models, the authors have developed a robust and flexible framework that can accurately recognize and interpret complex hand gestures from video data. This breakthrough has numerous applications, such as controlling virtual avatars in gaming or communicating with robots in manufacturing settings. As we continue to push the boundaries of artificial intelligence, innovations like ATM will pave the way towards a more intuitive and efficient interaction between humans and machines.
Computer Science, Computer Vision and Pattern Recognition
Efficient Event Data Processing via Sparse-Aware Solutions
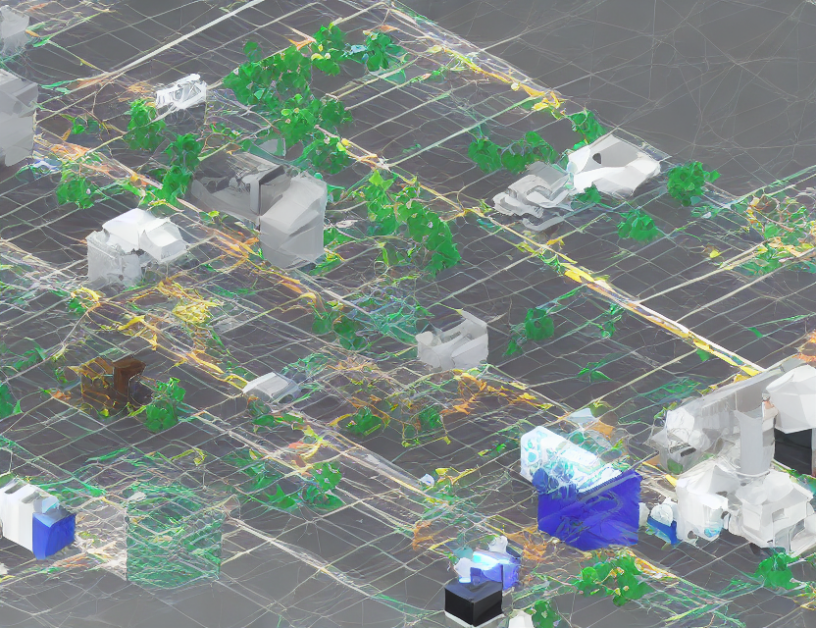