The article discusses the importance of explainability in artificial intelligence (AI) and machine learning (ML) models. The authors evaluate two different approaches to explaining AI models, a traditional method and a new method called "basic join." They analyze the performance of both methods on various datasets using metrics such as explanation error, area under the precision-recall curve, explanation time, and explanation sensitivity.
The results show that both methods have their strengths and weaknesses. The traditional approach has lower explanation error but requires more time for explanation creation, while the new method appears to be more robust in dealing with data noise. However, the basic join method performs better on some datasets.
The authors propose a methodology for evaluating explainability in AI models, focusing on the metrics proposed in their study. They also present a case study on all included datasets using the proposed metrics, with a focus on the explanation aspect.
Analogy: Explainability in AI models is like a chef explaining how they cooked a dish. Just as a chef wants to demonstrate the steps and ingredients involved in creating a dish, an AI model should be able to explain its decision-making process to provide transparency and trustworthiness.
Computer Science, Cryptography and Security
Exploring Explainability Metrics: A Comparison of Approaches in Deep Learning
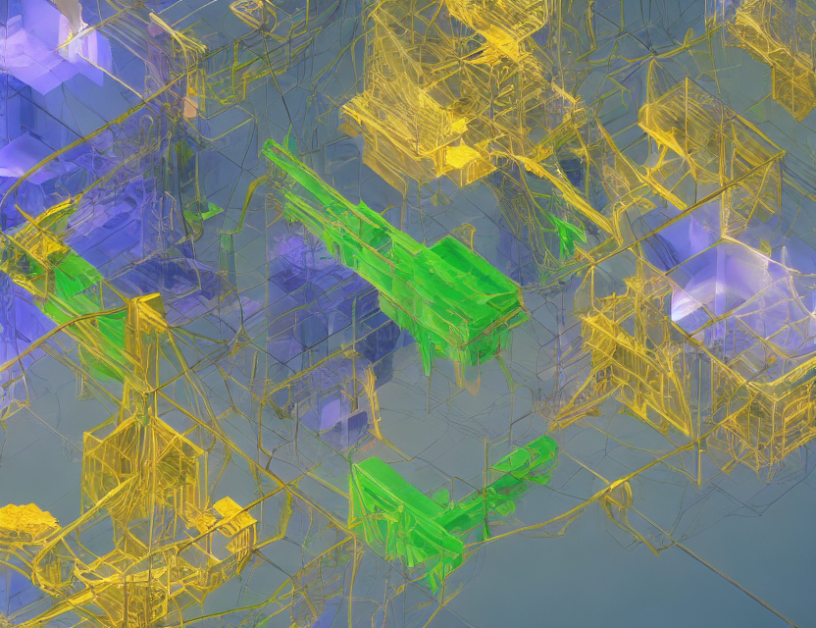