Hate speech detection is a crucial task to identify offensive language in digital media, and many researchers have proposed various approaches to tackle this problem. However, evaluating these systems remains challenging due to the lack of standardized evaluation metrics and datasets. This article aims to address this issue by providing a comprehensive survey of existing hate speech detection systems and their performance metrics.
The authors firstly discuss the different types of hate speech and the various approaches used in hate speech detection systems, including rule-based methods, machine learning models, and deep learning techniques. They then delve into the various evaluation metrics used to assess the performance of these systems, such as accuracy, F1 score, and macro F1 score.
To address the issue of lacking standardized datasets for hate speech detection, the authors propose a framework that combines in-context examples with the input data. They also discuss the importance of considering different learning paradigms, such as incremental and supervised learning, when evaluating these systems.
The article then summarizes the results of various recent studies on hate speech detection, highlighting their performance metrics and the datasets used. The authors also provide a comprehensive summary of the datasets used in these studies, including their size and label distribution.
Finally, the authors discuss some of the challenges and limitations of existing hate speech detection systems, such as dealing with imbalanced datasets and addressing the issue of false positives. They conclude by highlighting the need for further research to improve the accuracy and robustness of these systems.
In summary, this article provides a comprehensive overview of the state-of-the-art in hate speech detection systems, including their approaches, evaluation metrics, and results. It also discusses the challenges and limitations of these systems and highlights the need for further research to improve their accuracy and robustness.
Computation and Language, Computer Science
Exploring the Impact of Different Variations on Self-Ensembling Performance in NLP Tasks
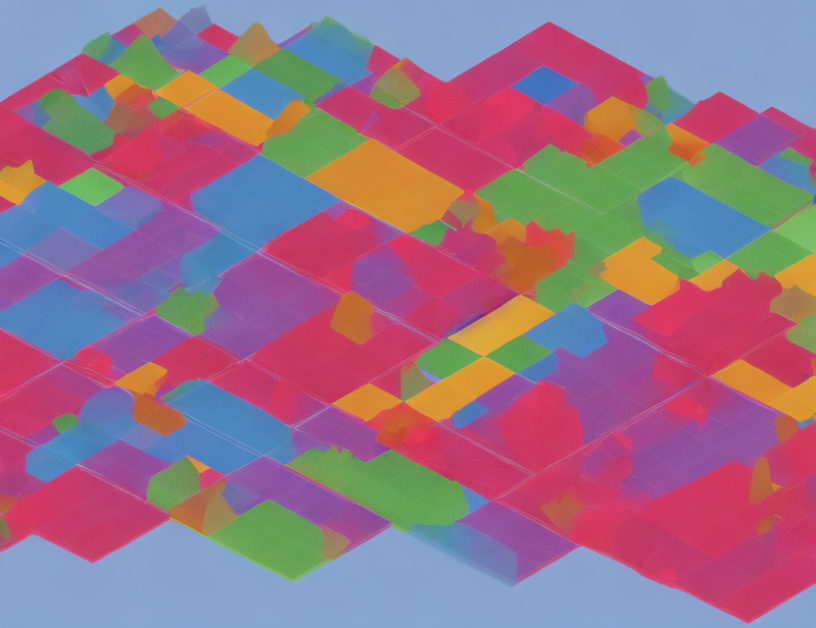