In the field of computer vision and natural language processing, researchers have been working on developing models that can understand and interpret images and text together. To achieve this goal, they need large datasets to train these models, but such datasets are rare. In this paper, the authors present Laion-5b, an open dataset containing over 2 million image-text pairs collected from various sources like websites, books, and documents. This dataset can be used to train next-generation image-text models that can recognize objects, understand context, and generate appropriate captions.
The authors explain that Laion-5b is different from other datasets in several ways. Firstly, it’s much larger than any existing dataset, making it ideal for training complex models. Secondly, the images are diverse and cover various categories like animals, buildings, and landscapes. Finally, the captions are generated using a novel approach that combines both visual and textual features to create more accurate descriptions.
To demonstrate the utility of Laion-5b, the authors conduct several experiments using state-of-the-art models. They show that these models can recognize objects in images with high accuracy and generate relevant captions that describe what’s happening in the image. The results suggest that Laion-5b can be used to train models that can understand both visual and textual information, paving the way for more advanced image-text understanding systems.
In conclusion, Laion-5b is a valuable resource for researchers working on developing next-generation image-text models. Its sheer size and diversity make it an ideal dataset for training complex models that can recognize objects, understand context, and generate accurate captions. With Laion-5b, the future of image-text understanding looks bright, with the potential to revolutionize various applications like image search, object detection, and visual question answering.
Computer Science, Computer Vision and Pattern Recognition
Large-Scale Dataset for Training Image-Text Models
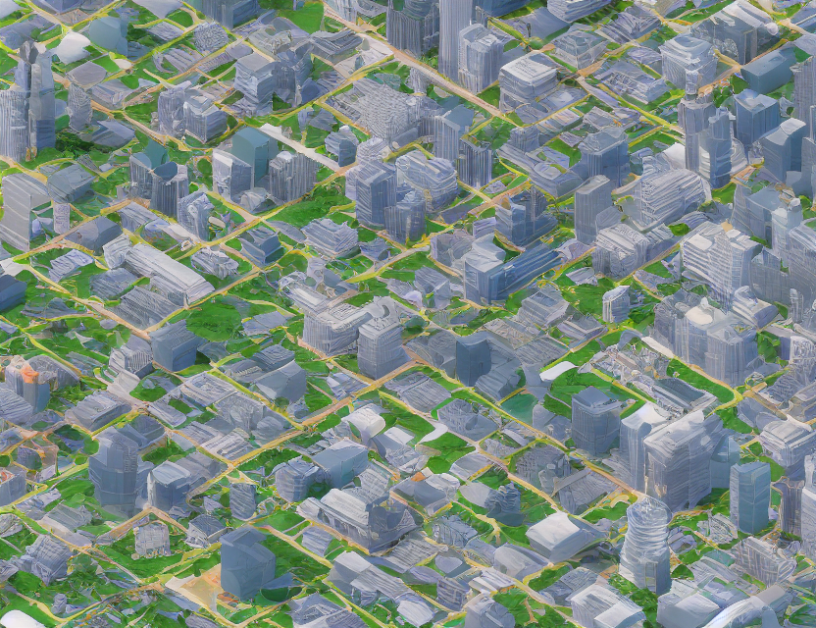