In this article, we delve into the world of Bayesian inference and explore how it has evolved over time. Bayesian inference is a statistical technique used to update probabilities based on new data or information. It’s like trying to estimate the probability of a coin tossing heads or tails after observing many tosses.
The authors, Gael M. Martin, David T. Frazier, and Christian P. Robert, trace the history of Bayesian inference from its early days in the 17th century to the modern era of computational power and machine learning. They highlight how advances in computing have enabled the development of more sophisticated algorithms for Bayesian inference, making it a powerful tool in various fields such as finance, biology, and physics.
One key concept in Bayesian inference is the Kullback-Leibler (KL) divergence, which measures the difference between two probability distributions. Imagining KL divergence as a thermometer that measures how hot or cold your favorite coffee is compared to your preferred temperature, can help illustrate its purpose.
The authors also discuss recent developments in Bayesian inference, such as the use of particle filters and Markov chain Monte Carlo (MCMC) methods. These techniques are like different cars on a racetrack, each with unique strengths and weaknesses, but all aimed at reaching the finish line of accurate probability estimation.
Another important aspect of Bayesian inference is the duality between control and inference, which can be likened to two sides of a coin. Control refers to making decisions based on probabilities, while inference refers to updating probabilities based on new data. This duality highlights the interplay between these two crucial aspects of Bayesian inference and underscores their shared goal of making informed decisions.
The article concludes by emphasizing the importance of considering both the theoretical foundations and practical applications of Bayesian inference in any modern treatment of the subject. It’s like cooking a meal; you need to follow a recipe, but also consider your taste preferences for it to be enjoyable. By combining theoretical rigor with practical relevance, we can harness the full potential of Bayesian inference and unlock its secrets for tackling complex problems in various fields.
Computer Science, Machine Learning
Particle-Based Inference Techniques in Reinforcement Learning
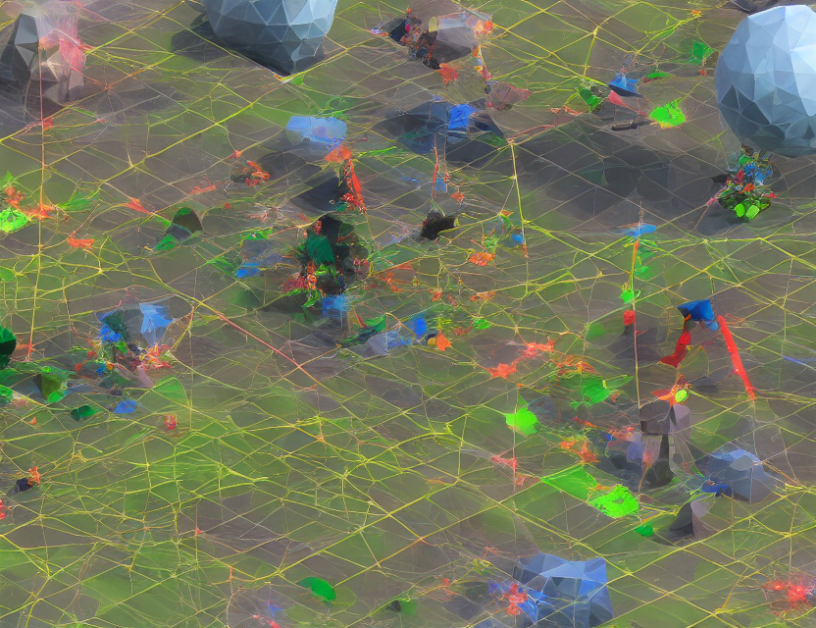