In recent years, there has been a growing interest in explainability in artificial intelligence (AI) research, particularly in the field of robotics. Explainability refers to the ability of AI systems to provide clear and understandable explanations for their decisions and actions. This is crucial in human-robot interaction as it helps humans understand why robots behave in certain ways, building trust and confidence in these systems.
The article discusses the importance of contextualizing human behavior and feedback when analyzing robot explainability. The authors found that using more context (i.e., more items in the antecedent) led to differences in robot explainability and gender emerging. They also showed that explainable robots can support situational awareness in human-machine teams, improve cooperative task efficiency, and reduce anxiety.
To illustrate their findings, the authors used a simple game example where humans played against an understandable or non-understandable robot. The interactions were manually transcribed and annotated with a video annotation software, resulting in 295 transactions for association rule mining. These transactions included items such as "RobotFeedback," "HumanFeedback," "Understandable," and "Female."
The authors emphasize that explainability is essential in robotics research to create robots that can effectively communicate their actions and decisions to humans. They also highlight the importance of considering context when analyzing robot explainability, as this can lead to more accurate and informative explanations.
In conclusion, the article stresses the significance of prioritizing explainability in AI research, particularly in human-robot interaction. By creating robots that can provide clear and understandable explanations for their actions, we can build trust and confidence in these systems, leading to more effective and efficient collaboration between humans and robots.
Contextualizing Human Behavior and Feedback in Robot-Human Interaction
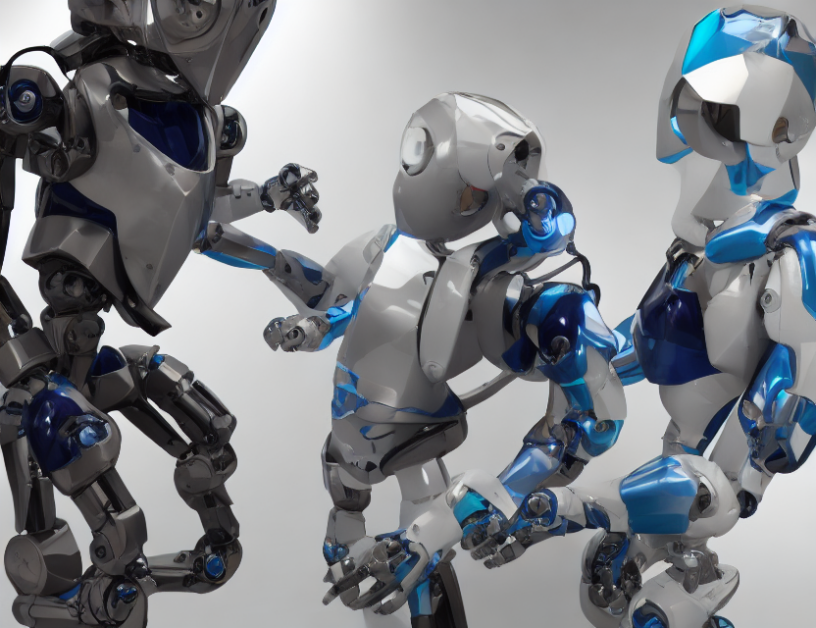