In this article, the authors explore how Large Language Models (LLMs) can help create structured datasets of equity-relevant information in various domains. These datasets are essential for understanding and mitigating biases, which are prevalent in society and often perpetuated by unstructured text data.
To create these datasets, LLMs can be used to identify and extract specific fields of information from raw text data. For instance, in healthcare, LLMs can help researchers extract measures of employment, housing, and insurance from clinical notes to understand how these factors impact patient outcomes. Similarly, in non-healthcare domains like law and criminal justice, LLMs can be applied to detect bias in trial transcripts.
The authors argue that LLMs present enormous potential to alleviate the burden of creating and maintaining structured datasets used to study inequity in multiple domains. By using LLMs to create these datasets, we can quantify disparities more accurately and develop policies to mitigate them.
To illustrate their point, the authors use an analogy: think of text data as an "artifact" created by a biased society. Just like how art historians examine artifacts to understand historical cultures, LLMs can examine text data to understand and mitigate biases.
In conclusion, this article highlights the potential of LLMs to create structured datasets that can help us better understand and address inequity in various domains. By democratizing the creation of these datasets, we can accelerate progress towards a more just society.
Computer Science, Computers and Society
Detecting Bias in Healthcare Texts: AI’s Role in Mitigating Disparities
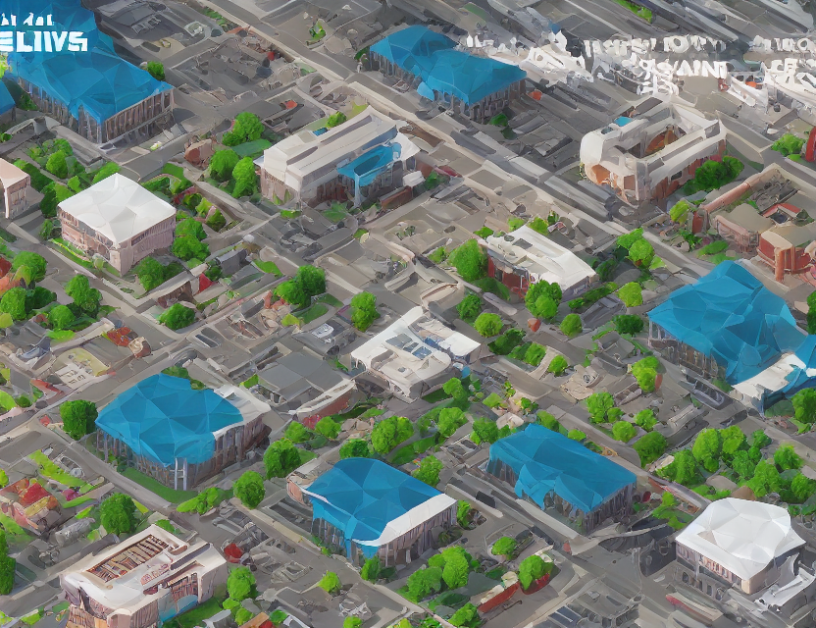