In this article, we explore the potential of latent spaces to improve the quality and controllability of generative models. Latent spaces are like a treasure trove of hidden knowledge that can be used to create more accurate and diverse images. By understanding how these spaces work, we can develop new techniques to manipulate them in a targeted manner, allowing us to eliminate unwanted features or attributes from generated images.
One approach is called "Feature Aware Similarity Thresholding" (FAST), which uses user-provided positive and negative samples to detect and remove undesirable features. This method relies on the latent space’s remarkable ability to represent intricate semantic features in a compact and meaningful way. In essence, we are using the latent space as a tool to understand what makes an image desirable or not, and then modifying it accordingly.
The article highlights the importance of regulating generative models and addressing concerns about offensive or injurious content. Machine unlearning is one solution, but traditional approaches often require access to model parameters and architectural details, which may not be feasible in many scenarios. By harnessing the power of latent spaces, we can develop new methods that can selectively remove unwanted knowledge without compromising the functionality of pre-trained models.
In summary, this article explores how latent spaces can help us create more robust and controllable generative models. By leveraging these hidden layers of knowledge, we can eliminate undesirable features, improve image quality, and develop new techniques for regulatory compliance. The use of machine unlearning and the latent space provides a promising solution for managing generative models in a responsible and efficient manner.
Computer Science, Machine Learning
Filtering as Weak Unlearning for Black-Box Generative Models
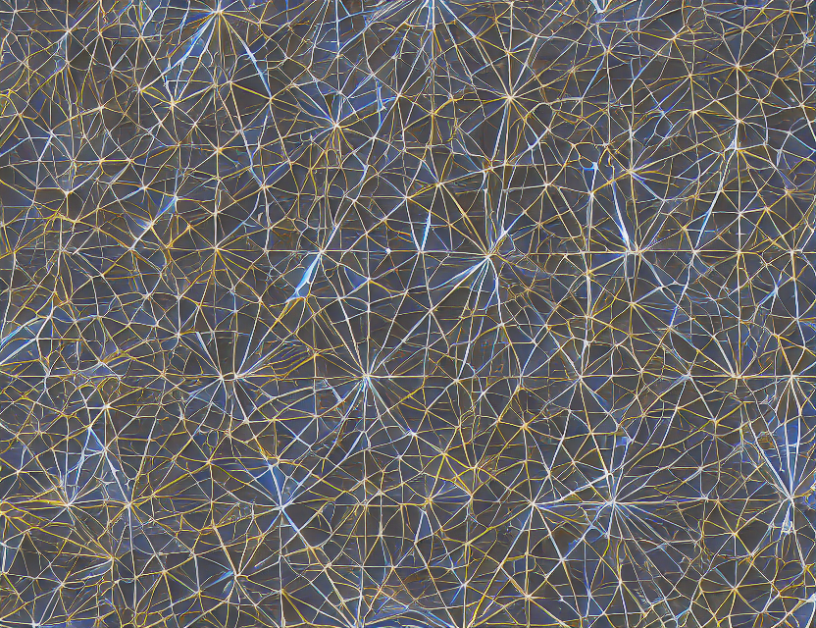