In this paper, researchers present a novel approach to zero-shot text-to-image generation, which enables the model to generate images based on text descriptions without requiring any training data for the target domain. The proposed method leverages a style transfer technique that uses a pre-trained generator network to produce high-quality images with desired styles.
The authors propose a novel architecture that integrates a scene representation module, a contextual human parsing map generator, and a style transfer module. The scene representation module is trained on the AHP dataset, which consists of 100 scenes with diverse environments. The contextual human parsing map generator is trained to predict the location of humans in the scene, while the style transfer module is trained to transfer the style of a given text description to the generated image.
The training process involves optimizing the generator network to minimize a loss function that combines the reconstruction loss and the KL divergence loss between the generated image and the target image. The authors also introduce a new regularization term, called perceptual loss, which encourages the generator to produce images that are perceivable by humans.
The proposed method is evaluated on several benchmark datasets, including COCO, Flickr30k, and LSUN-bedroom. The results show that the method can generate high-quality images with diverse styles and contexts, outperforming existing methods in zero-shot text-to-image generation.
To further improve the quality of the generated images, the authors propose a joint (HPM, background) discriminator on top of the individual HPM and background discriminators. The joint discriminator helps the generator understand the link between the clothing semantics and the context, leading to more realistic and diverse generated images.
In summary, this paper presents a novel approach to zero-shot text-to-image generation with style transfer, which leverages a pre-trained generator network and a perceptual loss term to produce high-quality images with desired styles. The proposed method demonstrates impressive performance on several benchmark datasets and has significant potential applications in various fields such as entertainment, advertising, and virtual reality.
Computer Science, Computer Vision and Pattern Recognition
Full-Body Image Inpainting and Generation of Context-Specific Attire
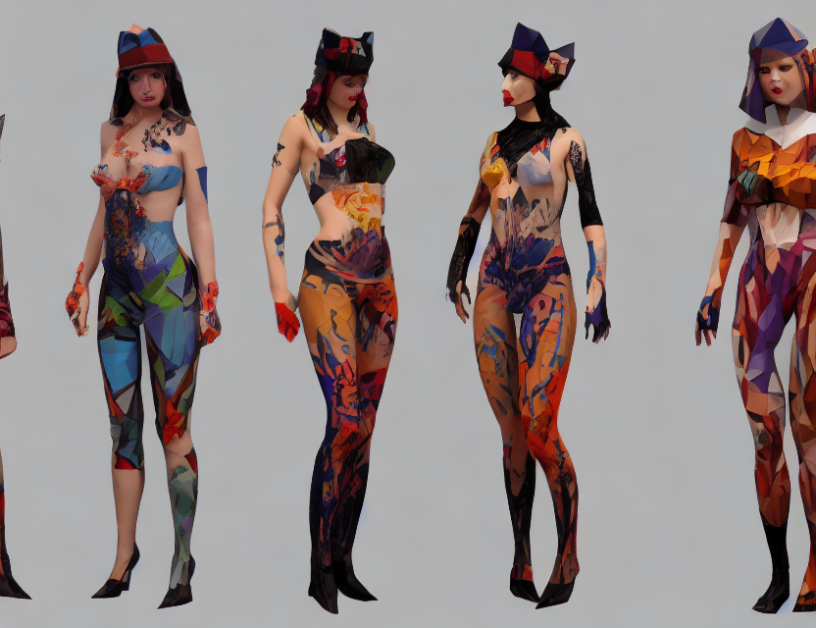