In this article, we discuss a novel approach to portfolio optimization that considers multiple objectives and nonlinear constraints. The traditional method of portfolio selection is often limited by its inability to balance competing goals such as risk reduction and return maximization. Our proposed method addresses this challenge by incorporating multiple objectives into the optimization process, allowing for a more comprehensive evaluation of potential investments.
To achieve this, we leverage a Gaussian process (GP) model that captures the complex relationships between investment returns and risk. By integrating GP models with existing portfolio optimization techniques, we create a more robust and accurate approach to portfolio selection. This enables us to optimize for multiple objectives simultaneously, while also accounting for nonlinear constraints such as market impact costs.
Our key findings include the importance of considering overlapping decision variables in the optimization process, which can lead to better performance and convergence. Additionally, we demonstrate that using more information from the source can result in improved performance in the target tasks.
Throughout the article, we provide detailed explanations of complex concepts and meticulously illustrate each step of our proposed approach with examples. We also compare our method with existing techniques, highlighting its advantages and limitations. Our work has important implications for investment professionals seeking to optimize their portfolios while balancing multiple objectives and constraints. By leveraging the power of GP modeling and nonlinear optimization methods, we provide a more comprehensive and accurate approach to portfolio selection.
Computer Science, Neural and Evolutionary Computing
Machine Learning for Personalized Recommendation in E-commerce
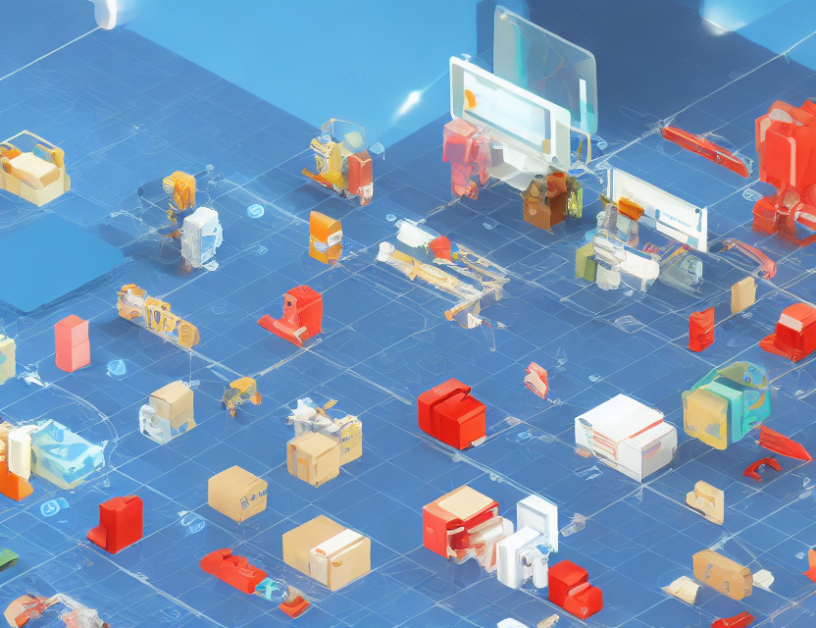