In this article, we present a new model that helps us understand how opinions evolve within groups with strong divisions. The model is based on two key principles: polarization maximization and bounded confidence. By leveraging these principles, the model can explore complex social interactions and opinion dynamics in a more accurate way than previous models.
To begin with, let’s consider a group of people with diverse opinions. When they interact with each other, their opinions can either be reinforced or challenged, leading to changes in their beliefs. Our model takes this process into account by introducing a polarization function that measures the degree to which individuals align with one another.
The key insight of our model is that polarization maximization leads to more accurate predictions of opinion dynamics. In other words, the model aims to increase the difference between the most extreme opinions in the group. This is achieved by adjusting the communication between agents based on their degree of similarity or diversity.
The model also takes into account bounded confidence, which means that individuals are less likely to change their minds if they are surrounded by people with similar views. By incorporating this aspect, the model can better capture how polarization emerges in groups over time.
In summary, our model provides a valuable framework for understanding the complex dynamics of opinion evolution within polarized groups. By leveraging the principles of polarization maximization and bounded confidence, it can accurately predict how opinions will change over time and why individuals tend to gravitate towards more extreme views.
Computer Science, Multiagent Systems
Polarization Opinion Model Inspired by Bounded Confidence Communications
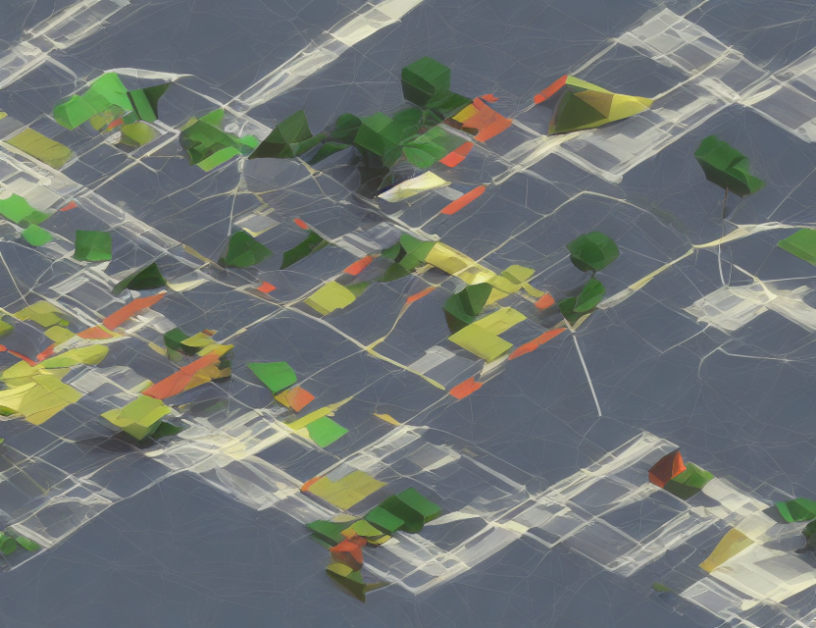