In this article, we delve into the realm of machine learning (ML) and deep learning (DL) technologies that have taken center stage in today’s technology landscape. These powerful tools enable us to uncover novel patterns in complex data, leading to groundbreaking results across various disciplines. However, as these technologies become increasingly sophisticated, a pressing challenge emerges – the need for interpretability.
Imagine a black box that hides the inner workings of deep learning models from us. Although these models are incredibly powerful, their complexity and obscurity make it difficult to understand how they arrive at their conclusions. This lack of transparency can erode confidence in ML and DL systems, particularly in high-stakes environments.
To address this challenge, we propose a novel approach that balances simplicity and performance. By expanding the dimension of the input data strategically, we can enhance the system’s ability to handle complex classifications while maintaining interpretability. This approach emerges as both the most parsimonious and effective solution in the literature.
As we double the number of classes from five to ten, the challenges intensify, adding layers of sophistication to our exploration. The model must provide finer discernment, requiring it to manage increased complexity with precision. Our proposed approach successfully navigates these demands while maintaining its performance standards.
In summary, this article delves into the intricacies of ML and DL technologies, highlighting their potential for groundbreaking results across various disciplines. However, as these technologies become increasingly sophisticated, the need for interpretability grows more pressing. Our proposed approach balances simplicity and performance, providing a solution that is both effective and easy to understand. By expanding the dimension of the input data strategically, we can enhance the system’s ability to handle complex classifications while maintaining transparency, thereby mitigating the challenges that arise with increasing complexity.
Computer Science, Machine Learning
Unlocking Machine Learning’s Potential: A New Approach to Robustness and Resilience
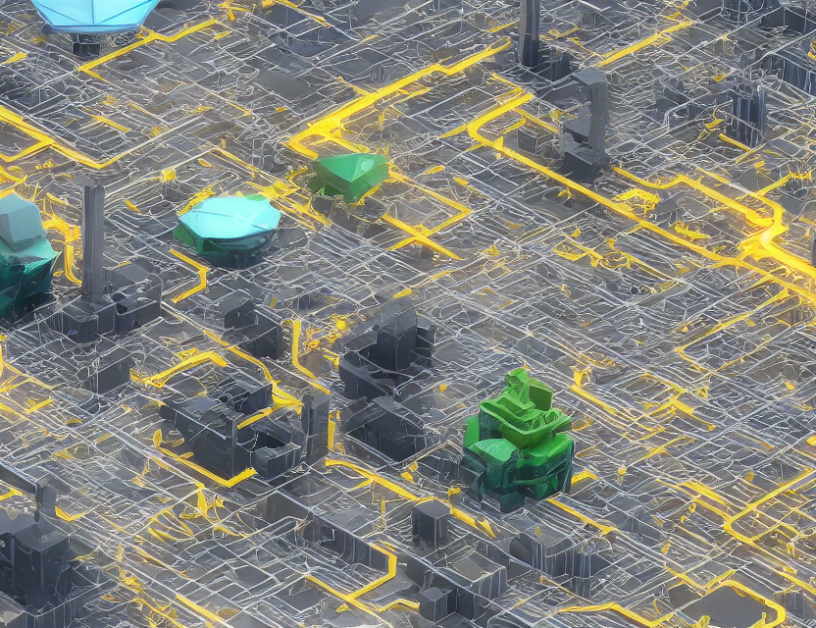