In this article, researchers explore the potential of using convolutional neural networks (CNNs) to improve the sorting of smartphone components for recycling. The team created a dataset of labeled images of various smartphone components, which they used to train their CNN model. They achieved high accuracy in identifying and separating different component types, such as batteries, screens, and circuit boards.
The authors note that the current method of recycling electronic waste (e-waste) is often inefficient and lacks proper documentation, leading to significant amounts of hazardous materials ending up in landfills or being illegally dumped. They argue that the use of CNNs could help improve the accuracy and efficiency of e-waste recycling by enabling the automatic separation and classification of components.
To explain their approach, the authors use an analogy to food sorting: just as a fruit and vegetable sorting machine can quickly and accurately sort different types of produce, a CNN can be trained to recognize and separate different smartphone components based on their visual features. They also highlight that their approach is not limited to smartphones but could be applied to other electronic devices as well.
The study’s findings have important implications for the recycling industry, as they demonstrate that CNNs can help improve the efficiency and accuracy of e-waste recycling. The authors hope that their work will contribute to the development of more sustainable and efficient methods for managing electronic waste in the future.
In conclusion, this article provides a promising solution for improving the sorting of smartphone components for recycling using CNNs. By leveraging the power of machine learning, researchers have shown that it is possible to efficiently separate and classify different component types, paving the way for more sustainable e-waste management practices.
Computer Science, Computer Vision and Pattern Recognition
Efficient Recycling of Electronic Waste: A Comprehensive Review
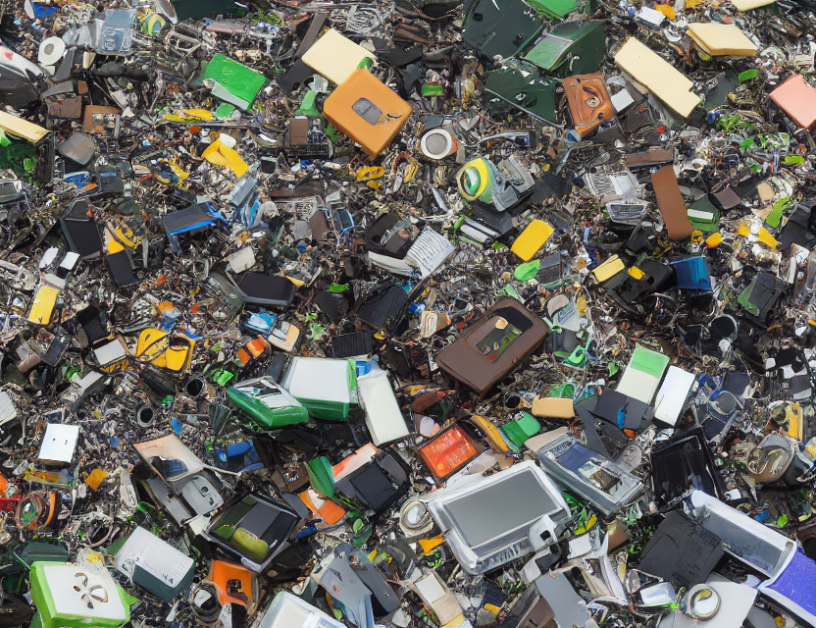