In the field of video captioning, proposals are generated to represent an arbitrary shape of a video. However, existing methods have limitations, such as selecting one proposal from a large set of proposals or relying on pre-trained models. To address these issues, this article introduces a Gaussian-based approach that generates a small set of proposals with enhanced expression ability.
The proposed method generates Gaussian mixture proposals, which can effectively represent an arbitrary shape of a video. Unlike previous methods, this approach does not require selecting one proposal from a large set or relying on pre-trained models. Instead, it iteratively refines proposal confidence scores to prevent biased grounding results.
The article demonstrates the effectiveness of the proposed method through experiments on the ActivityNet Captions dataset. The results show that the Gaussian-based approach outperforms existing methods in terms of proposal quality and expression ability.
In conclusion, this article introduces a novel approach to improving proposal quality in video captioning by leveraging Gaussian mixture proposals. The proposed method offers several advantages over existing methods, including improved accuracy and efficiency. By generating diverse proposals with enhanced expression ability, the method enables more accurate and informative video captioning.
Computer Science, Computer Vision and Pattern Recognition
Enhancing Expression Ability of Proposals for Video Sentence Prediction via Gaussian Mixture Approach
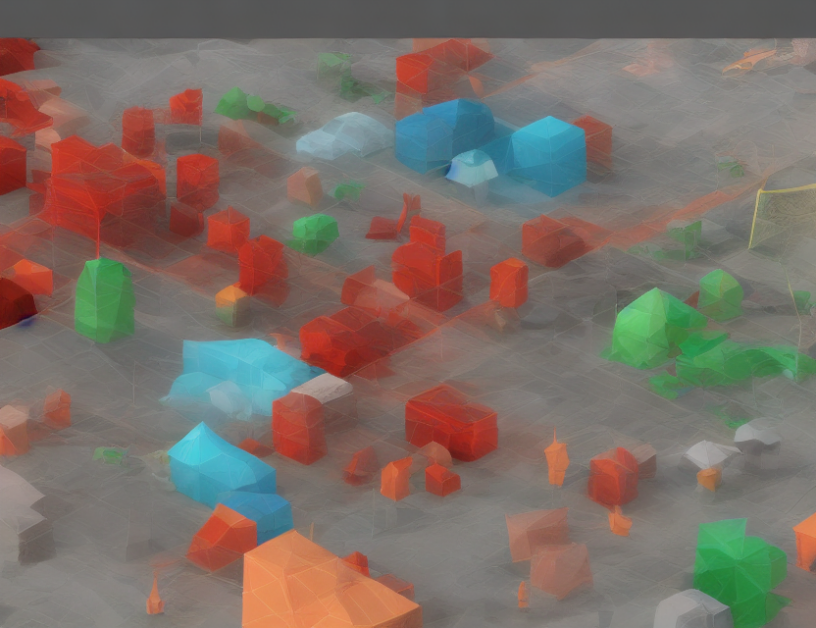