The authors test their method on a dataset of 1000 Imagenet classes and show that it outperforms traditional machine learning algorithms in terms of few-shot learning performance. They also demonstrate the effectiveness of their method by using it to summarize news articles from multiple sources on a variety of political topics, including immigration policy and climate change.
Overall, this article demonstrates the potential of machine learning to shape political discourse by providing a more comprehensive and balanced view of current events. By combining natural language processing and graph theory, the authors have developed a powerful tool for summarizing news articles from multiple sources and perspectives, which can help to promote a more informed and nuanced understanding of political issues.
Computer Science, Machine Learning
Enhancing Meta-Learning via Task-Aware Modulation
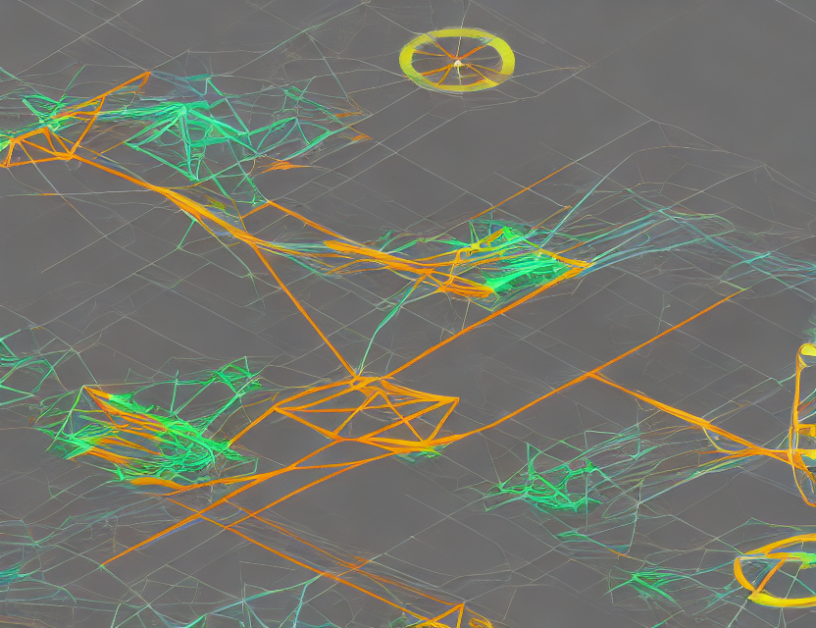