In this research paper, the authors propose a novel approach to crowd counting using both visual and textual information. They introduce CLIP, an image encoder and text encoder that work together to extract embeddings for both image and text, which are then used to count the number of specified objects in an image. The authors experiment with different combinations of encoder layers and find that the shallow encoder layers do not perform well due to their limited ability to capture meaningful patch-level information. They choose features from the 7th, 8th, and 9th encoding layers and incorporate them into the 2nd, 3rd, and 4th decoding layers.
The authors also explore the influence of context prompts on their method, using various templates to provide context for the image and text embeddings. They find that incorporating context into their model improves its performance and allows it to better understand the meaning of the visual and textual information it is processing. Overall, the authors demonstrate the effectiveness of their approach and highlight its potential for real-world applications in computer vision and natural language processing.
Computer Science, Computer Vision and Pattern Recognition
Optimizing Semantic-Conditioning Techniques for Image Captioning: A Comprehensive Analysis
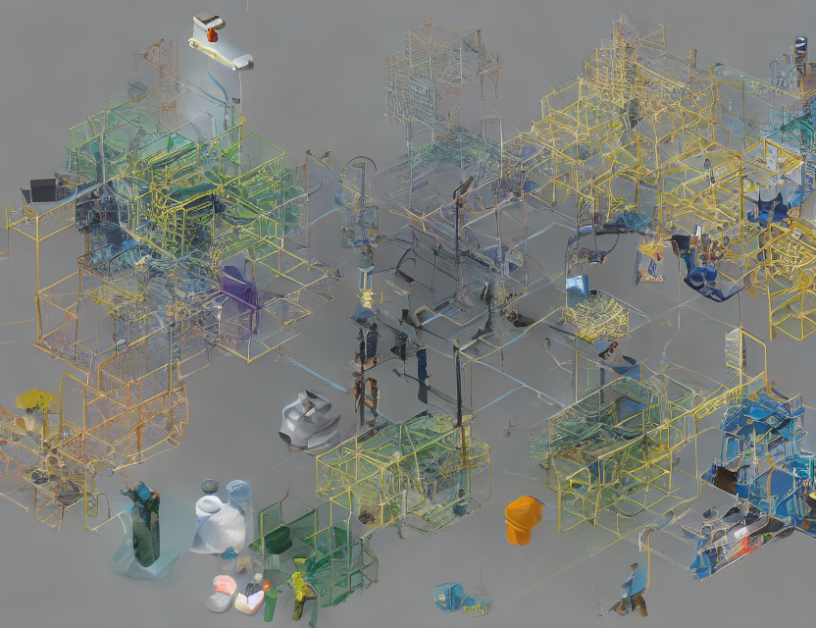