In this article, we present PanGu-Draw, a cutting-edge image generation model that sets a new standard in both quality and efficiency. Developed by Zhiyu Jin, Xuli Shen, Bin Li, and Xiangyang Xue, PanGu-Draw leverages advanced techniques to enhance the generation process, resulting in images of unparalleled fidelity.
Achieving this level of quality without sacrificing efficiency was a top priority for the authors. To achieve this, they utilized a novel approach that divided the training process into two stages: one dedicated to structural outlines and another to textual details. This division allows for more efficient training while maintaining flexibility in data quality.
The PanGu-Draw model relies on a combination of innovative techniques to reduce redundant memory usage, including replacing traditional attention with Flash Attention, employing mixed-precision training, and using gradient checkpointing. These methods enable the model to fit within the memory of a single Neural Processing Unit (NPU), allowing parallelism to be applied only in the data scope and avoiding model sharding among NPUs, as well as reducing inter-machine communication overhead.
In addition, the authors collected images from various sources, including Noah-Wukong, LAION, and others, and filtered them based on CLIP score, aesthetic score, watermark presence, resolution, and aspect ratio. They also developed an advanced comprehension ability of large language models (LLMs) to align users’ succinct inputs with the detailed inputs required by the model, further enhancing generation quality.
The PanGu-Draw model demonstrates remarkable efficiency, reducing data preparation time by 48% and resource consumption by 51%. With its impressive capabilities, PanGu-Draw has the potential to revolutionize the field of image generation and pave the way for new applications in various industries.
Computer Science, Computer Vision and Pattern Recognition
Training-Free Diffusion Model Adaptation for Variable-Sized Text-to-Image Synthesis
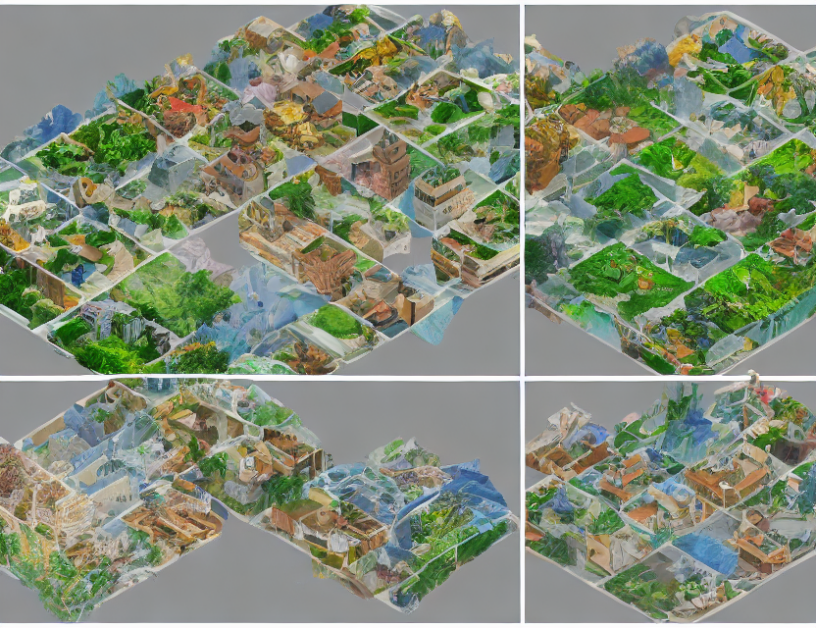