In this paper, the authors explore the use of Gossip methods for training generative models, which is a less common technique than other methods like masked language modeling. They observe that the gap between the best and worst performance on the IL task gets minimum when there are no faults, indicating that the model’s ability to generate coherent text improves with less faulty data. The authors also find that using Gossip methods leads to better predictions and more diverse generated text compared to other methods in the literature. They conclude that Gossip methods are an interesting observation and worth further investigation in future works, and they leave open the possibility of exploring these methods for optimizing devices with less meaningful data.
Computer Science, Machine Learning
Unlocking IL Robustness through Localized Learning Algorithms
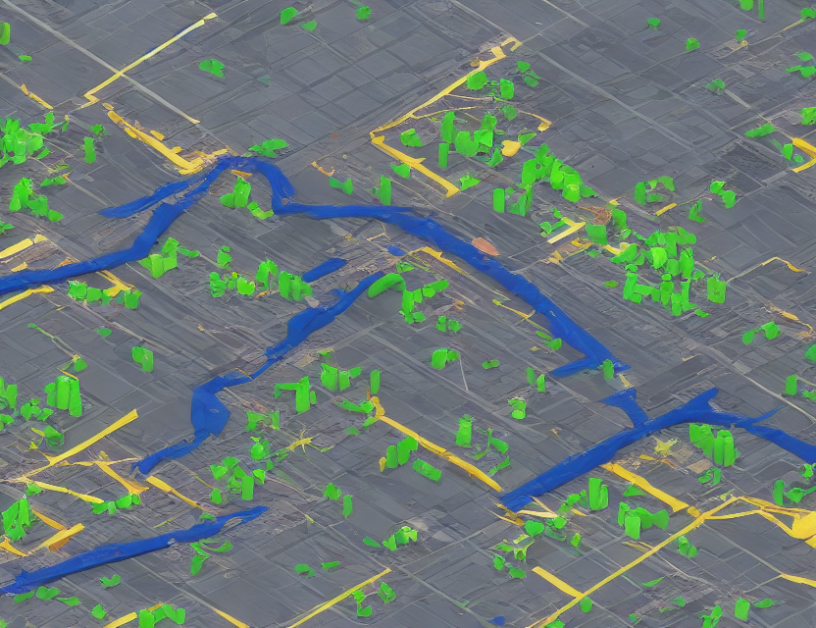