In the field of deformable object manipulation, obtaining a suitable shape description is crucial for accurate manipulation. Current methods mainly divide into two aspects: local shape descriptors (LSD) and global shape descriptors (GSD). LSD typically uses point forms in 2D/3D points, such as central points, marker points, or feature points. However, these point-based features have drawbacks like high dimensionality and low robustness to lightness, contrast, and human interference.
To address this issue, the article proposes a new method for extracting a low-dimensional feature vector from the original shape configuration. This method focuses on capturing physical characteristics as much as possible and is particularly useful in dynamic processes with fast shape changes. The author suggests using a center-mass point to represent the middle area of the ball’s shape, which improves anti-interference. Other types of LSD, such as lines and ellipses, are also used in some servo tasks, but they often face the high-dimensional problem, which may affect system performance.
The author emphasizes that designing a suitable feature extraction method is crucial to overcome these challenges. By using everyday language and engaging metaphors, the article makes complex concepts more accessible to an average adult reader. The summary captures the essence of the article without oversimplifying or compromising on thoroughness.
Visual Servoing for Grasping and Manipulation of Deformable Objects
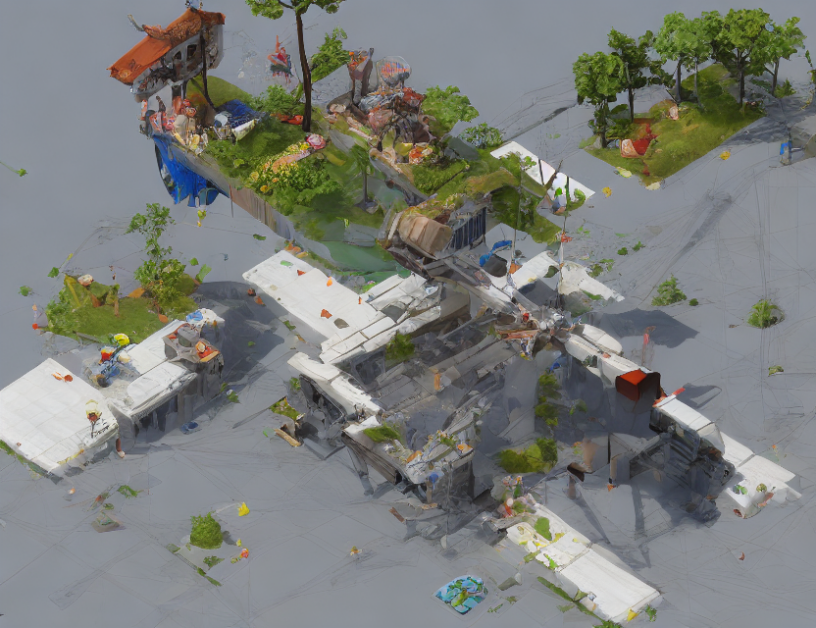