Memorizing Convolution Blocks (MC-Blocks): Unlocking Dynamic Correlations for Efficient Time Series Analysis
Time series analysis is a crucial task in various fields, including finance, healthcare, and environmental monitoring. However, dealing with long sequences of data can be challenging due to the complexity of capturing dynamic correlations across different scales. To address this challenge, researchers have introduced Memorizing Convolution Blocks (MC-Blocks), a key component in learning dynamic correlations and denoising representations.
The MC-Blocks hierarchical structure allows for extracting information and denoising at multiple scales. Each level represents the overall information of the sequence from a specific perspective, enhancing the efficiency of information processing and memory utilization. The shared parameters and memory in MC-Blocks at the same level facilitate this process, ensuring that the information is passed seamlessly between levels.
The article presents various experiments conducted on different datasets to demonstrate the effectiveness of MC-Blocks. The results show that MC-Blocks outperform traditional methods in terms of accuracy and computational efficiency. The authors also provide a detailed analysis of the performance comparison on different datasets, highlighting the best results.
One of the most significant advantages of MC-Blocks is their ability to capture long-term dependencies. Unlike traditional methods that rely solely on short-term information, MC-Blocks incorporate knowledge from multiple scales, enabling more accurate forecasting. This is achieved through the use of non-linear transformations, which help to reveal hidden patterns in the data.
In conclusion, Memorizing Convolution Blocks represent a significant breakthrough in time series analysis. By leveraging the power of hierarchical structures and shared parameters, MC-Blocks enable more accurate and efficient forecasting, making it possible to unlock dynamic correlations that were previously hidden. This innovative approach has far-reaching implications for various fields, including finance, healthcare, and environmental monitoring, and is poised to revolutionize the way we analyze time series data in the future.
Computer Science, Machine Learning
Improving Time Series Forecasting with Attention-Based Models
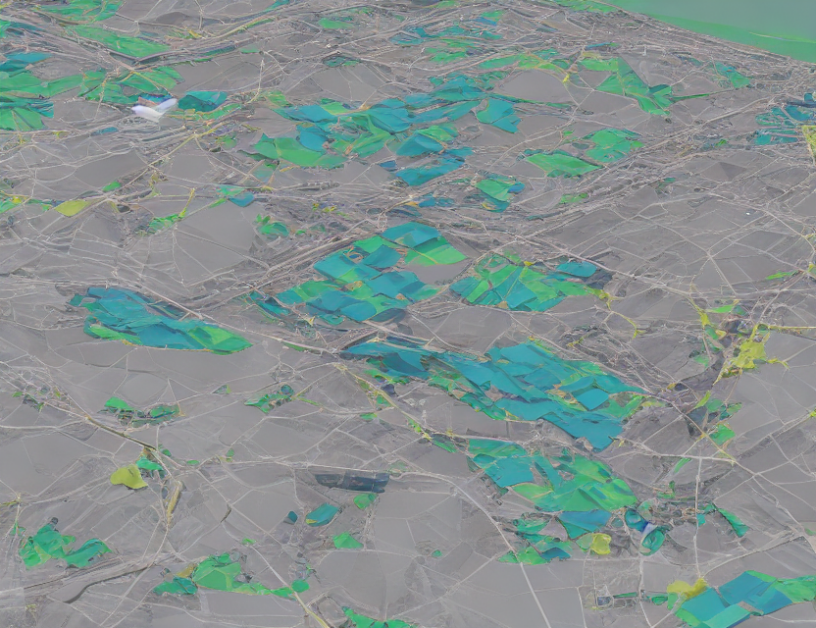