In recent years, there has been a surge in the quality and diversity of generated content, primarily due to the use of Diffusion Models (DMs) trained on vast amounts of data. DMs have enabled inexperienced users to produce impressive results using only a textual prompt. However, while these models are intuitive, they often fall short in capturing precise nuances, leading to potential mismatches with the user’s intentions.
To address this issue, researchers have been extending these paradigms to videos. The majority of current methods rely on a text prompt to guide the editing process and introduce new techniques to improve the smoothness and temporal consistency across generated frames.
One approach is to use a noise prior for video diffusion models, which preserves the user’s correlation between frames. Another method is tokenflow, which considers multiple concepts in a single image and extracts them in a way that is consistent with the user’s intentions.
Furthermore, researchers have proposed methods that use a text-driven layered image and video editing approach, such as Text2live, which enables high-resolution video synthesis with latent diffusion models. These models align your latents to produce videos that are both visually appealing and coherent.
In summary, the article discusses how researchers have been developing new techniques to improve text-to-image and video editing tasks using diffusion models. The approaches aim to capture the user’s intentions more accurately, leading to higher quality and more personalized results. By leveraging these techniques, users can create impressive videos with ease, without requiring extensive knowledge of computer vision or machine learning.
Computer Science, Computer Vision and Pattern Recognition
Enhancing Video Editing with Diffusion Models: A New Frontier
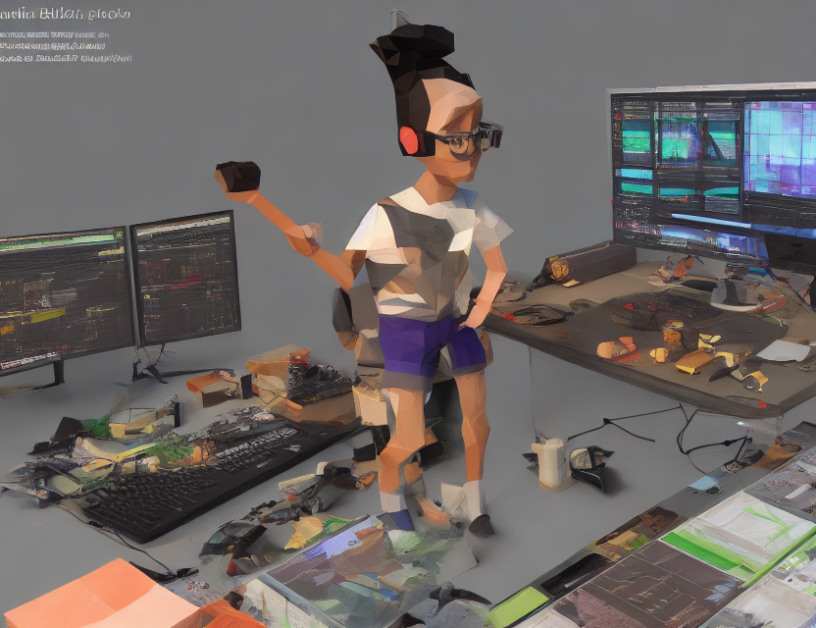