One approach is to fine-tune LLMs like GPT4 on a large dataset like iNaturalist, which has over 10 million images. Another strategy is to use CLIP, a popular image captioning model, to generate texts for a subset of categories in the CUB dataset. Researchers also explore different ways of using CLIP’s similarity scores as an indicator of visibility, such as pairing texts above a certain threshold or using max pooling at the instance level.
Overall, fine-grained image captioning is a challenging task that requires careful consideration of various factors to achieve accurate and informative descriptions. By developing and refining LLMs like InstructBLIP and MiniGPT4, researchers are working towards improving the quality of these descriptions and enhancing our ability to understand and interpret visual data.
Computer Science, Computer Vision and Pattern Recognition
Fine-tuning CLIP for Novel Fine-grained Image Captioning
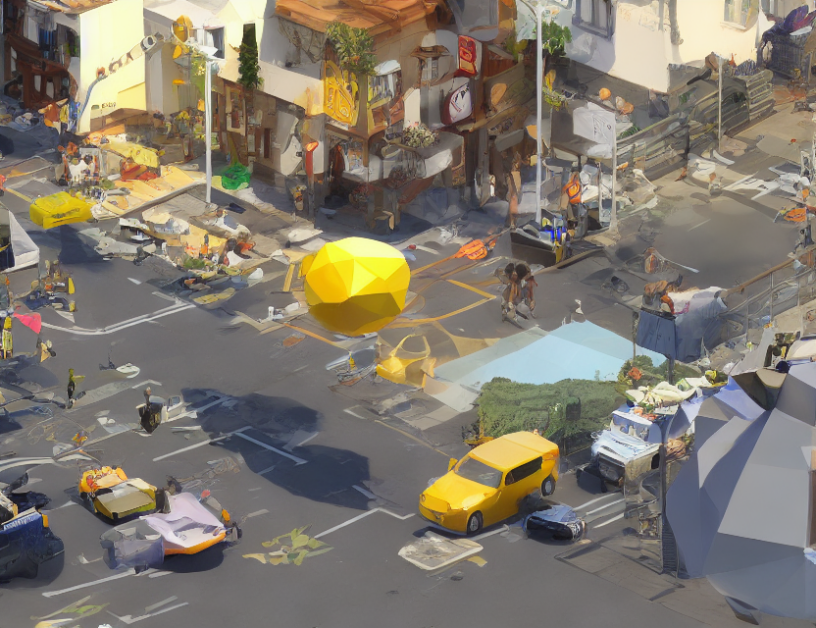