In this article, we present OptFlow, a novel method for real-time point cloud processing that achieves state-of-the-art results in flow estimation while providing faster inference times than existing techniques. Our approach leverages a simple and efficient algorithm that doesn’t require complex annotations or training data, making it suitable for applications such as autonomous driving.
We evaluate OptFlow on four major datasets and show that our method consistently outperforms other non-learning-based approaches across all datasets while also delivering the fastest inference time. Additionally, we provide a detailed analysis of our algorithm’s performance over a range of timesteps on the KITTI dataset, demonstrating optimal performance at the 600th iteration with inference times of approximately 2.3 seconds for 2048 points and 3.1 seconds for 8192 points.
Our method is designed to handle high-density point clouds without imposing range constraints, allowing for more accurate flow estimation. We also compare our approach to prior work in the field, demonstrating its competitiveness in terms of performance, speed of execution, and model complexity. Overall, OptFlow represents a significant advancement in real-time point cloud processing, enabling the development of more sophisticated applications such as autonomous vehicles.
Computer Science, Computer Vision and Pattern Recognition
OptFlow: Real-time Scene Flow Estimation with State-of-the-art Results
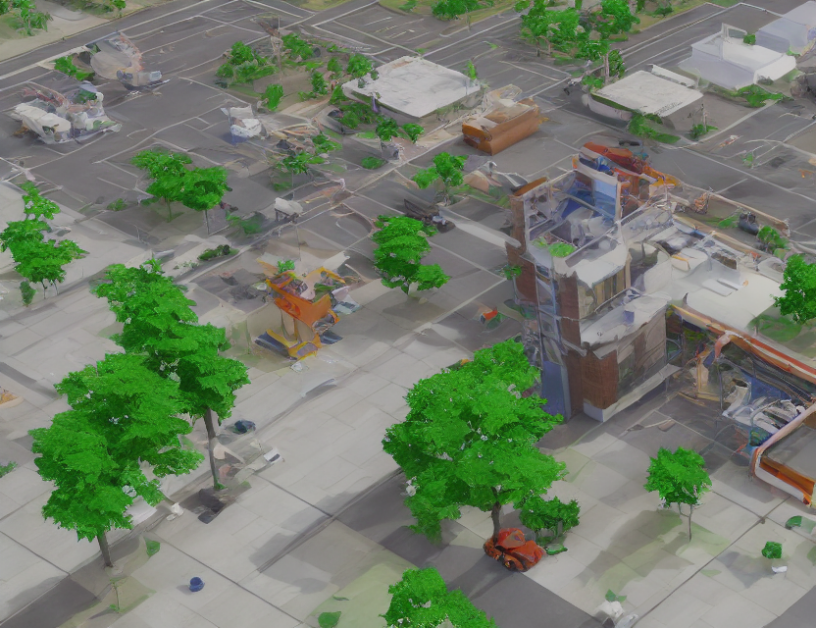