In this article, the authors propose a new architecture called Powerformer to enhance the traditional Transformer’s ability to handle sectional power system power flow adjustment. The proposed approach incorporates section information into the attention matrix and utilizes a multi-factor mechanism to enable more effectively state representations.
The authors explain that the traditional Transformer has limitations when dealing with sectional power systems, as it only considers the self-attention of each head without considering the relationship between heads. To address this issue, Powerformer employs a section-adaptive attention mechanism that takes into account all factors, rather than just one head. This is achieved by using a softmax function on the attention matrix, which allows the model to consider all factors simultaneously.
The authors also propose a new multi-factor mechanism that combines multiple representations from different heads, enabling more effectively state representations. This is done by recombining a new attention matrix, which is formed by combining different section-adaptive attention matrices, and then applying a softmax function on the dimensions of these factors.
To evaluate the effectiveness of Powerformer, comprehensive case studies are conducted using various comparison methods and popular adjustment baselines. The results demonstrate that Powerformer outperforms other methods in terms of power flow accuracy and computational efficiency.
Finally, the authors conduct ablation studies to enhance understanding of their proposed method. These studies show that the section-adaptive attention mechanism and multi-factor mechanism are essential components of Powerformer, and that combining these mechanisms leads to better performance.
In conclusion, Powerformer is a novel Transformer-based architecture that improves the ability of traditional Transformers to handle sectional power system power flow adjustment. By incorporating section information into the attention matrix and utilizing a multi-factor mechanism, Powerformer enables more effectively state representations, leading to better performance and computational efficiency.
Computer Science, Machine Learning
Attentive Representations for Image Set Classification
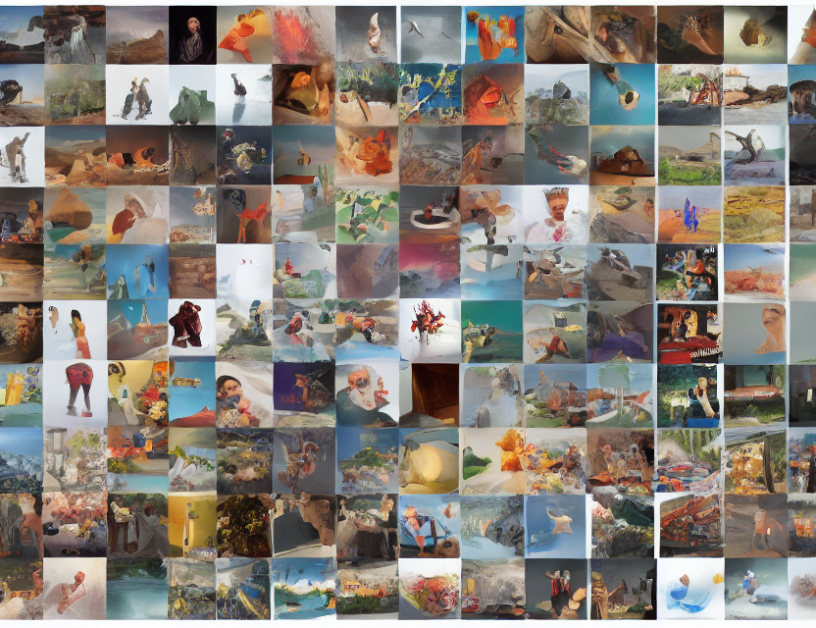