In this article, we explore how to enhance NeRF (Neural Radiance Fields) representations by elevating them to a 4D space. This involves adopting two mechanisms that help separate shape and motion within the latent space. We use a GAN-NeRF model that incorporates these mechanisms, which leads to better disentanglement of shape and motion. Our approach leverages the inherent editability within the latent space and enables us to set the facial geometry control p to zero, resulting in 3D-view consistent and temporally coherent editing.
To begin with, NeRF is a technique that allows us to represent 3D scenes using a neural network. However, these representations are limited to a 3D space, which doesn’t capture the spatio-temporal information of the scene. To address this limitation, we propose elevating NeRF to a 4D space by separating shape and motion within the latent space.
To achieve this separation, we use two mechanisms. The first mechanism consists of a canonical space and a definition space, as outlined in various studies [24, 25, 27, 28]. This mechanism helps separate shape from motion in the latent space. The second mechanism involves conditioning the original NeRF on time-related variables [44, 47, 56], which helps capture the temporal information of the scene.
We adopt these two mechanisms in our dynamic NeRF representation and incorporate them into a GAN-NeRF model [45, 48]. The GAN-NeRF model leverages the inherent editability within the latent space to enable us to set the facial geometry control p to zero. This results in 3D-view consistent and temporally coherent editing.
To further enhance our representation, we use a Latent Mapper [26] that offers a short inference time of 75ms when pre-trained for a particular text prompt. The backbone of the StyleClip is the 2D StyleGAN [16], which we replace with the Omniavatar Generator. This allows us to control the editing process more precisely, as all expressions are deformed with respect to the canonical space.
In summary, our article proposes elevating NeRF representations to a 4D space by separating shape and motion within the latent space using two mechanisms. We adopt these mechanisms in a GAN-NeRF model that leverages the inherent editability within the latent space for 3D-view consistent and temporally coherent editing. Our approach offers improved disentanglement of shape and motion, enabling more precise control over the editing process.
Computer Science, Computer Vision and Pattern Recognition
Elevating Video Editing to 4D Space with Dynamic NeRF
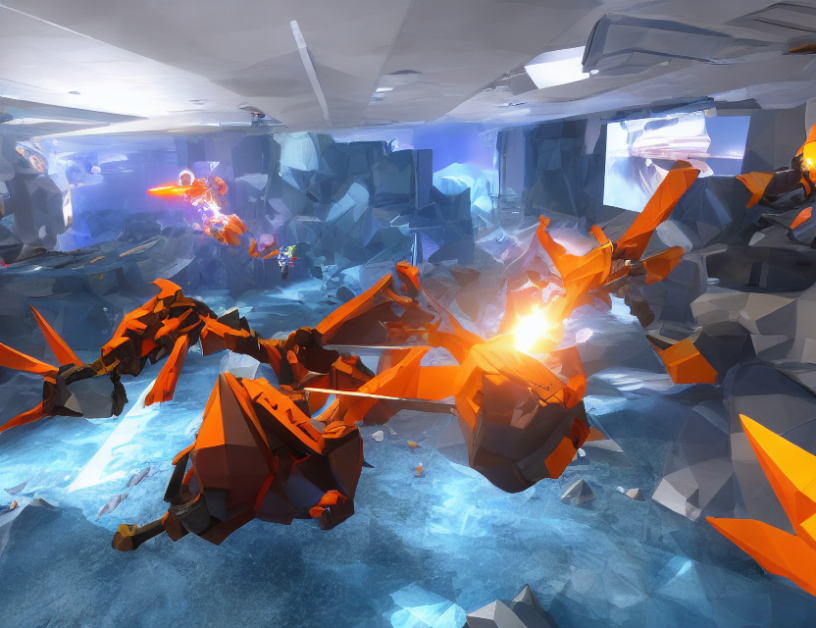