In this article, we delve into the concept of abstraction in causal inference, which is the process of identifying cause-and-effect relationships between variables. Abstraction is crucial because real-world data often lacks the necessary level of detail to accurately determine causality. The authors explain that humans understand their surroundings through abstract concepts, and this ability is also essential for modern intelligent systems.
The discussion begins by defining causal variables, which are the variables of interest in a given context. However, these variables may not always align with the features of the data, and therefore, two sets of variables are defined: VL (low granularity) and VH (high granularity). The constitutional hierarchy arises from organizing variables based on their level of granularity, allowing for a proper abstraction of causal properties across different levels.
The article highlights the importance of choosing the appropriate level of granularity when constructing causal diagrams. This decision depends on various factors, including non-causal relationships not being visible at higher levels of granularity, admissibility of the resulting clustering, queries of interest being answerable and identifiable, and coarseness of the result.
To demystify complex concepts, the authors use everyday language and engaging metaphors. For instance, they explain that variables can be organized like a collection of building blocks, where each block represents a different level of granularity. They also compare causal abstraction to a puzzle, where the goal is to find the right pieces that fit together to form a complete picture.
Overall, this article provides a concise and comprehensive overview of abstraction in causal inference, making it accessible to an average adult reader. By using relatable analogies and avoiding technical jargon, the authors successfully demystify complex concepts and capture the essence of the topic without oversimplifying.
Computer Science, Machine Learning
Learning Abstract Causal Generative Models for Higher-Level Concepts
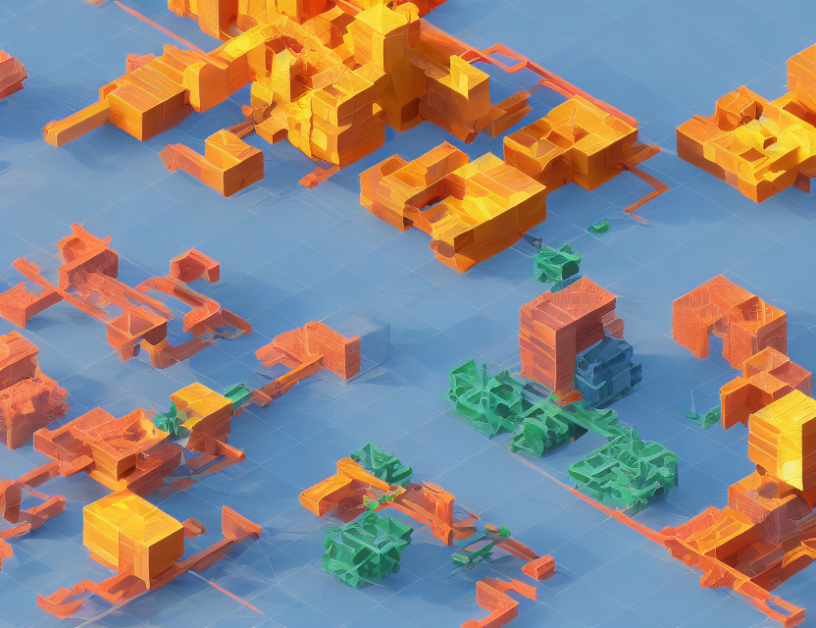