The authors then describe the training process for AMBR, which involves fine-tuning BART models on each dataset (XSum and SAMSum) and using InfoLM as a utility function. They also explain that they generate 64 samples for each dataset to evaluate the model’s performance.
Next, the authors discuss their evaluation methodology, which involves comparing the generated summaries with human-generated summaries from two datasets (XSum and SAMSum). They show that AMBR outperforms existing methods on both datasets.
Finally, the authors conclude by highlighting the contributions of their work and future directions for research in abstractive sentence summarization. They note that while their model performs well, there is still room for improvement, particularly in terms of generating more diverse and informative summaries.
Overall, this paper provides a detailed description of a neural attention model for abstractive sentence summarization, including the proposed approach, training methodology, and evaluation metrics. The authors provide a clear and concise explanation of their work, making it accessible to readers who may not be familiar with the field of natural language processing.
Artificial Intelligence, Computer Science
Minimum Bayes Risk Automatic Speech Recognition
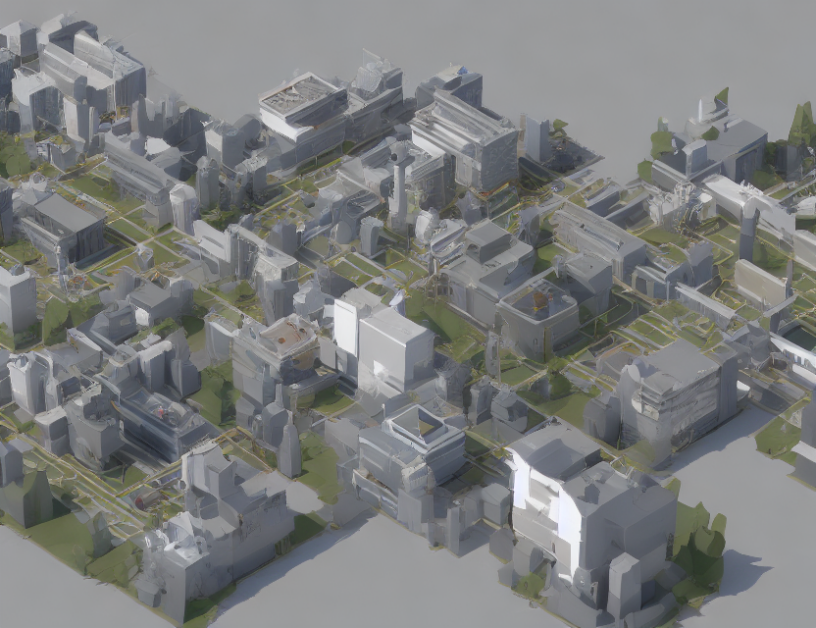